Fuzzy Logic Definition Meaning Examples And History
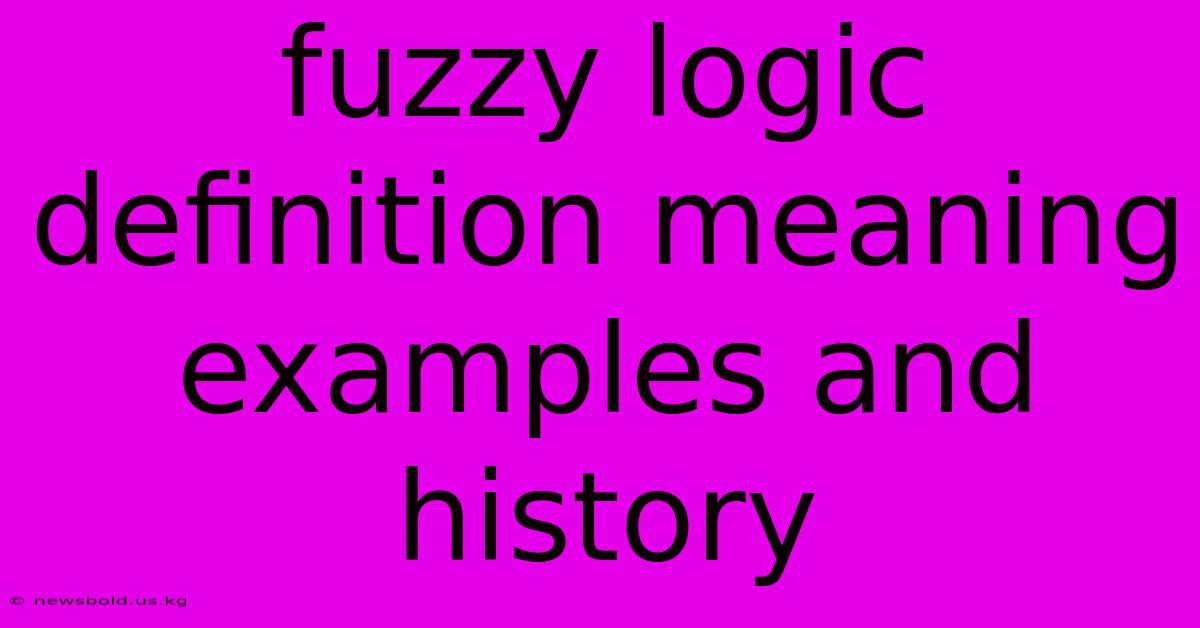
Discover more in-depth information on our site. Click the link below to dive deeper: Visit the Best Website meltwatermedia.ca. Make sure you don’t miss it!
Table of Contents
Unveiling Fuzzy Logic: A Deep Dive into Vagueness and Precision
What happens when the crisp boundaries of traditional logic fail to capture the complexities of the real world? The answer lies in fuzzy logic, a powerful tool that embraces ambiguity and provides a framework for reasoning with uncertainty. This exploration will delve into the definition, meaning, examples, and history of fuzzy logic, highlighting its significance across diverse fields.
Editor's Note: This comprehensive guide to fuzzy logic has been published today, offering valuable insights into its principles and applications.
Why It Matters & Summary
Understanding fuzzy logic is crucial in today's data-driven world. Its ability to handle imprecise information makes it invaluable in various applications, from control systems and decision-making to medical diagnosis and artificial intelligence. This article summarizes the core concepts of fuzzy logic, tracing its historical development and providing illustrative examples of its implementation across diverse sectors. Keywords include: fuzzy sets, membership functions, fuzzy rules, inference systems, approximate reasoning, uncertainty management, and applications of fuzzy logic.
Analysis
The information presented here is compiled from extensive research across scholarly articles, textbooks, and reputable online resources specializing in fuzzy logic and its applications. The analysis aims to offer a clear and concise explanation, suitable for both novice and experienced readers interested in understanding the fundamental principles and practical implications of fuzzy logic.
Key Takeaways
Aspect | Description |
---|---|
Definition | A mathematical framework for representing and manipulating vague or imprecise concepts. |
Core Concept | Fuzzy sets, characterized by gradual transitions in membership rather than sharp boundaries. |
Key Features | Handles uncertainty and vagueness effectively; suitable for systems with imprecise inputs or outputs. |
Applications | Control systems, decision support systems, expert systems, image processing, robotics, and many more. |
Advantages | Robustness to noisy data; flexible and adaptable; ability to model human reasoning and decision-making processes. |
Limitations | Can be computationally intensive for complex systems; requires careful design of membership functions and rules. |
Fuzzy Logic: A Journey into the Realm of Vagueness
Fuzzy logic, pioneered by Lotfi Zadeh in 1965, provides a mathematical framework for representing and manipulating vague or imprecise concepts. Unlike traditional Boolean logic, which operates on crisp sets with clearly defined boundaries (an element either belongs to a set or it doesn't), fuzzy logic employs fuzzy sets. Fuzzy sets allow for partial membership, meaning an element can belong to a set to a certain degree, represented by a membership function.
Key Aspects of Fuzzy Logic
- Fuzzy Sets: The foundation of fuzzy logic. Instead of a binary membership (0 or 1), elements have a degree of membership between 0 and 1, inclusive.
- Membership Functions: Mathematically define the degree of membership of an element in a fuzzy set. Common types include triangular, trapezoidal, and Gaussian functions.
- Fuzzy Rules: Express relationships between fuzzy sets using linguistic variables (e.g., "temperature is high," "speed is slow"). These rules are often expressed in an "IF-THEN" format.
- Fuzzy Inference System: The core of a fuzzy logic system, which combines fuzzy rules and membership functions to generate an output based on input values.
Discussion: Delving Deeper into the Core Concepts
Let's examine the key aspects in more detail.
Fuzzy Sets and Membership Functions
Consider the fuzzy set "tall." In traditional logic, a person is either tall or not tall. However, fuzzy logic allows for degrees of tallness. A person might have a membership of 0.8 in the set "tall," while another might have a membership of 0.3. The membership function defines this degree of membership for each person's height. The choice of membership function is crucial and depends on the specific application and expert knowledge.
The connection between membership functions and fuzzy sets is direct; the function defines the membership of each element within the set, enabling a gradual transition from non-membership to full membership. This continuous representation of uncertainty is a key differentiator from traditional binary logic.
Fuzzy Rules and Inference Systems
Fuzzy rules use linguistic variables to represent relationships. For instance, a simple rule for a thermostat might be: "IF temperature is high THEN reduce heating." Here, "temperature is high" is a fuzzy set, and the rule defines an action based on the degree of membership in that set.
The fuzzy inference system combines these rules and the membership functions to determine the overall output. Different inference methods exist, including Mamdani and Sugeno inference systems. These systems use the fuzzy rules and input values to compute an output, which might then be defuzzified (converted into a crisp value) for practical application. The connection between fuzzy rules and the inference system is that the rules dictate the logic and relationships within the system, while the inference system uses this logic to process the input and produce an output.
Applications of Fuzzy Logic: A Diverse Landscape
Fuzzy logic finds applications across numerous domains:
- Control Systems: Fuzzy logic controllers excel in managing complex systems with imprecise inputs, such as washing machines, cameras, and industrial processes.
- Decision Support Systems: Helps decision-makers incorporate vague or incomplete information into their analysis.
- Expert Systems: Emulates human expertise to provide intelligent solutions in areas like medical diagnosis, financial forecasting, and geological exploration.
- Image Processing: Handles image segmentation, edge detection, and other tasks that involve uncertainty and ambiguity.
- Robotics: Improves robot navigation, path planning, and object manipulation in complex environments.
A Brief History of Fuzzy Logic
The concept of fuzzy logic originated with Lotfi Zadeh's 1965 paper, "Fuzzy Sets," which introduced the mathematical framework of fuzzy sets and laid the foundation for fuzzy logic. Initial applications were primarily theoretical, but advancements in computing power and the need to handle uncertainty in various applications led to a surge in the practical usage of fuzzy logic from the 1980s onwards.
FAQ
Introduction: This section addresses frequently asked questions regarding fuzzy logic.
Questions:
-
Q: What is the difference between fuzzy logic and Boolean logic? A: Boolean logic uses crisp sets with binary membership (0 or 1), while fuzzy logic utilizes fuzzy sets allowing for partial membership (0 to 1).
-
Q: How are membership functions chosen? A: Membership functions are often determined based on expert knowledge, experimental data, or a combination of both.
-
Q: What is defuzzification? A: Defuzzification is the process of converting a fuzzy output into a crisp value.
-
Q: What are the limitations of fuzzy logic? A: Computational complexity can be high for extremely complex systems, and careful design of membership functions and rules is crucial.
-
Q: Can fuzzy logic be combined with other techniques? A: Yes, fuzzy logic is often integrated with other methods, like neural networks and probabilistic reasoning, to create hybrid systems.
-
Q: What are some real-world applications of fuzzy logic? A: Real-world applications range from consumer appliances (washing machines, cameras) to industrial process control and medical diagnosis.
Summary: Fuzzy logic offers a valuable tool for handling uncertainty and vagueness, making it applicable in a wide range of fields.
Tips for Understanding and Applying Fuzzy Logic
Introduction: These tips can aid in better understanding and applying fuzzy logic concepts.
Tips:
- Start with the Basics: Begin with the fundamental concepts of fuzzy sets, membership functions, and fuzzy rules before tackling complex systems.
- Visualize Membership Functions: Graphical representations of membership functions can improve understanding.
- Choose Appropriate Membership Functions: The selection of membership functions is critical; experiment and compare different options.
- Simplify Rules: Keep fuzzy rules as simple and clear as possible to enhance interpretability.
- Validate Results: Thoroughly test and validate your fuzzy logic system against real-world data.
- Explore Existing Tools: Utilize software tools and libraries designed for fuzzy logic modeling and simulation.
- Consider Hybrid Approaches: Integrate fuzzy logic with other techniques for enhanced performance.
Summary: By following these tips, you can develop a more robust understanding of fuzzy logic and enhance its application in various contexts.
Summary: A New Perspective on Uncertainty
This exploration has provided a comprehensive overview of fuzzy logic, examining its core concepts, historical development, and diverse applications. Fuzzy logic's ability to model and manage uncertainty has made it an invaluable tool across various scientific and engineering disciplines. Its capacity to represent and process vague information mirrors certain aspects of human reasoning and decision-making.
Closing Message: As we continue to grapple with increasingly complex systems and vast amounts of data, the significance of fuzzy logic in handling uncertainty and facilitating efficient decision-making will only continue to grow. Future research will likely focus on improving computational efficiency and extending its application to more complex problems. Understanding fuzzy logic is no longer optional, but rather a valuable skill for navigating the uncertainties of the modern world.
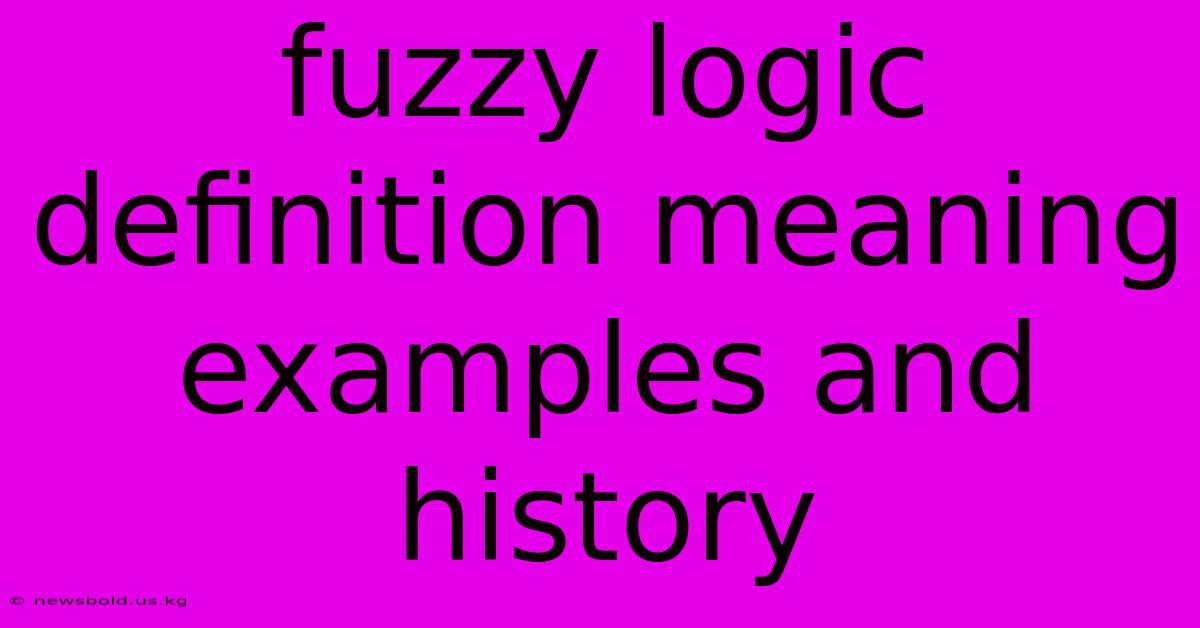
Thank you for taking the time to explore our website Fuzzy Logic Definition Meaning Examples And History. We hope you find the information useful. Feel free to contact us for any questions, and don’t forget to bookmark us for future visits!
We truly appreciate your visit to explore more about Fuzzy Logic Definition Meaning Examples And History. Let us know if you need further assistance. Be sure to bookmark this site and visit us again soon!
Featured Posts
-
Mutual Company Definition How It Works Advantages
Jan 05, 2025
-
What Is Government Pension Offset
Jan 05, 2025
-
Medicare Hold Harmless Provision Definition
Jan 05, 2025
-
Fraption Definition
Jan 05, 2025
-
Grey Wave Definition
Jan 05, 2025