Garch Model Definition And Uses In Statistics
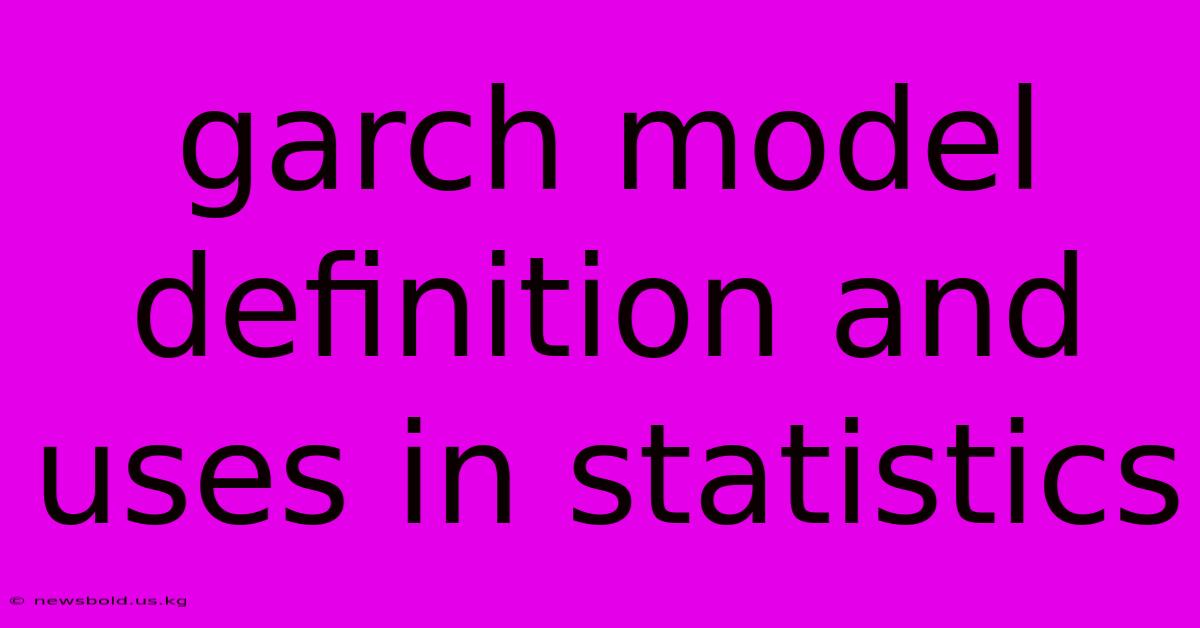
Discover more in-depth information on our site. Click the link below to dive deeper: Visit the Best Website meltwatermedia.ca. Make sure you don’t miss it!
Table of Contents
Unveiling GARCH: Modeling Volatility in Financial Time Series
What drives the fluctuating nature of financial markets? The answer lies, in part, within the realm of volatility modeling, and a powerful tool in this realm is the Generalized Autoregressive Conditional Heteroskedasticity (GARCH) model. This article explores the definition and applications of GARCH models in statistical analysis, revealing their significance in understanding and predicting market risk.
Editor's Note: This comprehensive guide to GARCH models has been published today, offering valuable insights into their practical applications in finance and beyond.
Why It Matters & Summary
Understanding and predicting volatility is paramount in finance, impacting investment strategies, risk management, and portfolio optimization. GARCH models provide a robust framework for capturing the time-varying nature of volatility, offering superior forecasting capabilities compared to traditional models that assume constant volatility. This article will delve into the core concepts of GARCH models, exploring their various specifications and applications, with a focus on their use in risk management and financial forecasting. Key terms explored include ARCH, GARCH(p,q), volatility clustering, and conditional variance.
Analysis
The analysis presented here draws upon established econometric literature and empirical studies demonstrating the effectiveness of GARCH models. The explanation of the model's mathematical formulation is simplified for accessibility while retaining the core principles. Examples are used to illustrate the application of GARCH models in different financial contexts.
Key Takeaways
Feature | Description |
---|---|
GARCH Definition | A statistical model capturing time-varying volatility in financial data, accounting for volatility clustering and persistence. |
ARCH Component | Models current volatility as a function of past squared residuals (shocks), representing the autoregressive nature of volatility. |
GARCH Component | Extends ARCH by including past conditional variances, providing a more comprehensive representation of volatility dynamics. |
Applications | Risk management, option pricing, portfolio optimization, trading strategies, forecasting, and analysis of macroeconomic variables. |
Limitations | Assumption of normality, potential for overfitting, and sensitivity to model parameterization. |
GARCH Model Definition
At its core, the GARCH model is a statistical tool used to model the volatility of a time series, particularly financial data like stock prices or exchange rates. Unlike simpler models that assume constant volatility (homoskedasticity), GARCH acknowledges that volatility changes over time (heteroskedasticity). This time-varying nature of volatility is often characterized by periods of high volatility followed by periods of low volatility, a phenomenon known as volatility clustering.
The GARCH model builds upon the Autoregressive Conditional Heteroskedasticity (ARCH) model. ARCH models express the conditional variance (volatility) of a time series as a function of past squared errors (residuals). However, ARCH models often require a high order to capture the persistent nature of volatility. GARCH models improve upon this by incorporating past conditional variances into the equation, making them more parsimonious and capable of modeling long-term volatility dependencies.
A common GARCH specification is the GARCH(p,q) model, where 'p' represents the number of lags of conditional variance and 'q' represents the number of lags of squared residuals. The general equation for a GARCH(1,1) model (the most frequently used) is:
σ²<sub>t</sub> = ω + αε²<sub>t-1</sub> + βσ²<sub>t-1</sub>
Where:
- σ²<sub>t</sub> is the conditional variance (volatility) at time t.
- ω is a constant term (positive).
- α is the coefficient of the squared residual at time t-1 (non-negative).
- β is the coefficient of the conditional variance at time t-1 (non-negative).
- ε<sub>t-1</sub> is the residual (error) at time t-1.
This equation indicates that current volatility depends on the previous period's squared residual (representing the impact of past shocks) and the previous period's volatility. The sum α + β represents the persistence of shocks, with values close to 1 indicating high persistence and slowly decaying volatility. The condition 0 ≤ α + β < 1 is necessary for the model's stationarity (meaning volatility doesn't explode over time).
Key Aspects of GARCH Models
Volatility Clustering
GARCH models effectively capture volatility clustering, a stylized fact observed in financial time series. Large shocks tend to be followed by other large shocks (positive or negative), and periods of high volatility are followed by periods of relatively high volatility, while periods of low volatility are followed by periods of low volatility. GARCH models explain this by allowing for the persistence of past shocks and volatility levels to impact future volatility.
Conditional Variance
The core of GARCH models lies in the concept of conditional variance. This refers to the variance of a variable given the information available up to a specific point in time. It's a measure of the uncertainty surrounding the variable, given what's known so far. Unlike unconditional variance, which is a single value for the entire time series, conditional variance varies over time reflecting changing market conditions.
Model Estimation
GARCH models are typically estimated using maximum likelihood estimation (MLE). MLE involves finding the parameter values (ω, α, β) that maximize the likelihood of observing the actual data given the model. Statistical software packages offer functions specifically designed for GARCH model estimation.
Uses of GARCH Models in Statistics
GARCH models find broad application in numerous statistical domains, with the most prominent being:
Risk Management
GARCH models are invaluable tools for financial risk management. By forecasting volatility, they allow investors and financial institutions to quantify and manage market risk more accurately. Value at Risk (VaR) calculations, a standard risk management technique, often leverage GARCH forecasts to estimate potential losses over a given time horizon and confidence level. Stress testing, a vital part of risk assessment, also benefits from incorporating GARCH-based volatility forecasts.
Option Pricing
Option pricing models, like the Black-Scholes model, rely on assumptions about volatility. However, volatility is not constant; GARCH models provide a framework for incorporating time-varying volatility into option pricing, leading to more realistic and accurate valuation of options. The stochastic volatility models, which are more complex, often incorporate GARCH-like principles to capture changing volatility over time.
Portfolio Optimization
Efficient portfolio optimization techniques, such as Markowitz's mean-variance optimization, require accurate estimates of asset returns and their covariances. GARCH models can be integrated into portfolio optimization to account for time-varying volatility and correlations, potentially leading to improved portfolio diversification and risk-adjusted returns.
Forecasting
GARCH models are effective forecasting tools for volatility. While they cannot predict the direction of price movements, their ability to forecast the magnitude of price fluctuations makes them valuable for anticipating market risk and adjusting investment strategies accordingly.
Macroeconomic Analysis
Beyond finance, GARCH models are used in macroeconomic analysis to model the volatility of economic variables like inflation, interest rates, and GDP growth. These models help understand the dynamics of macroeconomic shocks and their impact on economic stability.
FAQ
Introduction: This section addresses frequently asked questions about GARCH models.
Questions:
-
Q: What are the limitations of GARCH models? A: GARCH models assume that the error terms are normally distributed. This assumption may not hold in reality. Additionally, GARCH models can be sensitive to model specification (choice of p and q) and might overfit the data if not carefully selected.
-
Q: How does GARCH differ from ARCH? A: ARCH models only consider past squared residuals to explain volatility. GARCH models extend this by including past conditional variances, making them more efficient and capable of capturing persistent volatility.
-
Q: Can GARCH models predict the direction of market movements? A: No, GARCH models forecast volatility, not the direction of price changes. They provide information about the magnitude of potential price fluctuations, not the direction.
-
Q: What software can I use to estimate GARCH models? A: Many statistical software packages such as R, Python (with Statsmodels or arch libraries), EViews, and Stata offer functions for GARCH model estimation.
-
Q: What is the difference between GARCH(1,1) and GARCH(p,q)? A: GARCH(1,1) is a specific case of the general GARCH(p,q) model. GARCH(1,1) considers only one lag of the squared residual and one lag of the conditional variance. GARCH(p,q) allows for more lags, providing greater flexibility but increasing the risk of overfitting.
-
Q: How can I choose the optimal GARCH model order (p,q)? A: Model selection involves testing several orders (p,q) using information criteria like AIC (Akaike Information Criterion) or BIC (Bayesian Information Criterion). The model with the lowest AIC or BIC value is generally preferred, balancing model fit with complexity.
Summary: Understanding the limitations and practical considerations in selecting and applying GARCH models is crucial for accurate and reliable results.
Transition: Moving on, we will further explore the specific application of GARCH modeling in forecasting financial market volatility.
Tips for Using GARCH Models
Introduction: This section provides practical tips for effectively utilizing GARCH models.
Tips:
-
Data Preprocessing: Ensure your data is stationary before applying GARCH models. Non-stationarity can lead to misleading results. Common techniques include differencing or transformations.
-
Model Selection: Carefully select the optimal GARCH model order (p,q) using information criteria (AIC, BIC) to balance model fit and parsimony. Avoid overfitting.
-
Diagnostic Checks: After estimation, perform diagnostic checks to ensure model adequacy. This includes analyzing residuals for autocorrelation and normality.
-
Forecasting Evaluation: Evaluate the forecast accuracy of your model using appropriate metrics, such as RMSE (Root Mean Squared Error) or MAE (Mean Absolute Error).
-
Parameter Interpretation: Carefully interpret the estimated parameters (ω, α, β). The sum α + β indicates the persistence of shocks, with values closer to 1 signifying stronger persistence.
-
Consider Extensions: Explore GARCH extensions such as EGARCH (Exponential GARCH) or GJR-GARCH (Glosten, Jagannathan, and Runkle GARCH) if you suspect asymmetry in volatility responses to positive and negative shocks.
-
Out-of-Sample Testing: Always test the model's performance on out-of-sample data to ensure its generalizability and avoid overfitting.
Summary: Following these tips can lead to more accurate and reliable GARCH model implementations.
Transition: Let's conclude with a summary of the key findings and insights from this exploration of GARCH models.
Summary of GARCH Model Definition and Uses
GARCH models provide a powerful framework for modeling and forecasting time-varying volatility in financial and macroeconomic time series. Their ability to capture volatility clustering and persistence makes them crucial tools for risk management, option pricing, portfolio optimization, and forecasting. While limitations exist, careful model selection, diagnostic checks, and understanding the underlying assumptions are key for successful implementation.
Closing Message: This exploration of GARCH models underscores their importance in understanding and managing risk in dynamic environments. Further research into GARCH extensions and their integration with other modeling techniques will continue to advance our ability to understand and predict volatility in various fields.
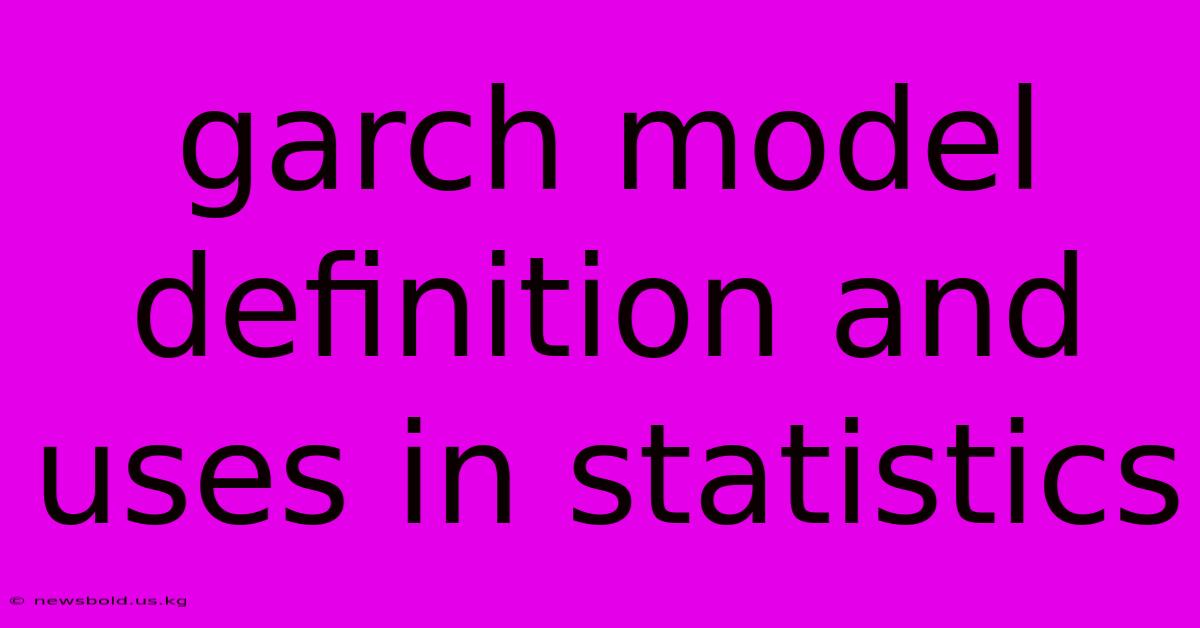
Thank you for taking the time to explore our website Garch Model Definition And Uses In Statistics. We hope you find the information useful. Feel free to contact us for any questions, and don’t forget to bookmark us for future visits!
We truly appreciate your visit to explore more about Garch Model Definition And Uses In Statistics. Let us know if you need further assistance. Be sure to bookmark this site and visit us again soon!
Featured Posts
-
Melt Up Definitionhow They Work Causes And Examples
Jan 05, 2025
-
Money Market Account Xtra Mmax Definition
Jan 05, 2025
-
Insurance Claim Definition
Jan 05, 2025
-
Greenspan Put Definition Examples Vs Fed Put
Jan 05, 2025
-
Institutional Deposits Corporation Idc Definition
Jan 05, 2025