Heteroscedasticity Definition Simple Meaning And Types Explained
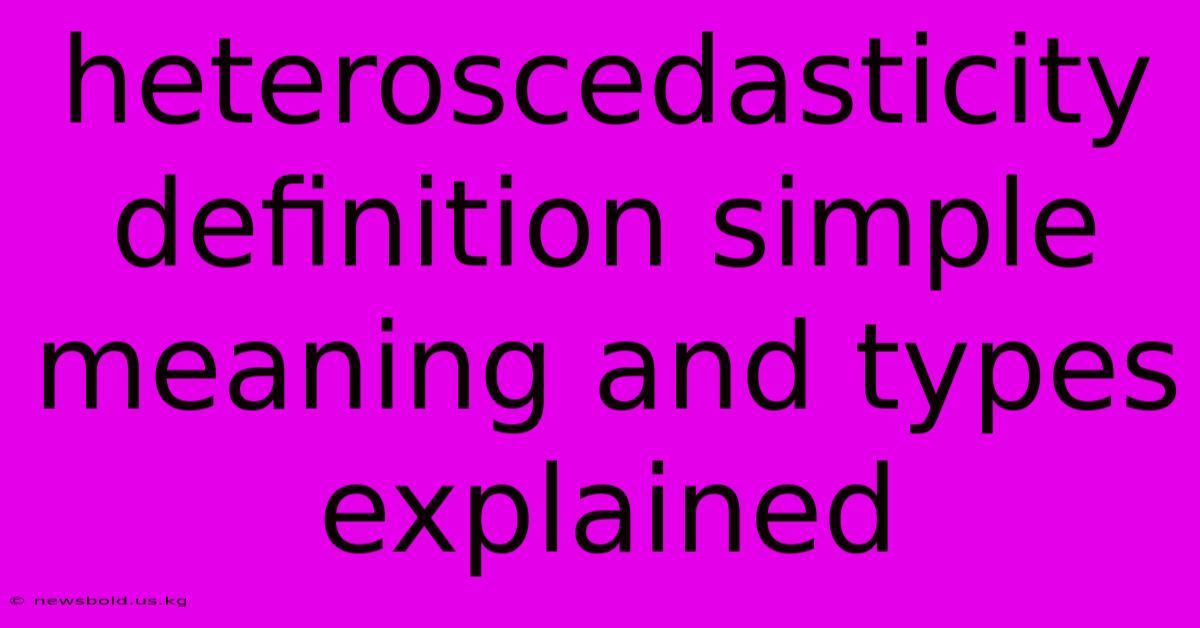
Discover more in-depth information on our site. Click the link below to dive deeper: Visit the Best Website meltwatermedia.ca. Make sure you don’t miss it!
Table of Contents
Unveiling Heteroscedasticity: Simple Meaning, Types, and Implications
What happens when the variability of your data isn't consistent across all observations? This inconsistency, a crucial concept in statistical analysis, is known as heteroscedasticity. Understanding its meaning and implications is vital for accurate modeling and reliable inferences.
Editor's Note: This comprehensive guide to heteroscedasticity was published today.
Why It Matters & Summary
Heteroscedasticity, meaning "unequal scatter," significantly impacts the reliability of statistical analyses, particularly regression models. Failing to address it can lead to inaccurate standard errors, unreliable hypothesis tests, and inefficient parameter estimates. This article will explore the simple meaning of heteroscedasticity, its different types, detection methods, and strategies for mitigation. Keywords include: heteroscedasticity, homoscedasticity, regression analysis, variance, standard errors, statistical modeling, data analysis, residual plots, weighted least squares.
Analysis
This guide utilizes a combination of theoretical explanations, practical examples, and visual representations to illustrate the concept of heteroscedasticity. The analysis draws upon established statistical principles and best practices for data analysis. The aim is to provide a clear and comprehensive understanding of heteroscedasticity, enabling readers to identify and address it effectively in their own statistical analyses.
Key Takeaways
Feature | Description |
---|---|
Heteroscedasticity | Unequal variance of data points across the range of predictor variables. |
Homoscedasticity | Equal variance of data points across the range of predictor variables. |
Detection Methods | Residual plots, Breusch-Pagan test, White test |
Consequences | Inaccurate standard errors, inefficient parameter estimates, unreliable hypothesis tests |
Mitigation | Weighted least squares, transformations of variables |
Let's delve deeper into the subject.
Heteroscedasticity: A Detailed Exploration
Heteroscedasticity, in its simplest form, refers to the unequal spread or dispersion of data points around a regression line. In other words, the variability of the dependent variable is not constant across different values of the independent variable(s). This contrasts with homoscedasticity, where the variance is constant.
Key Aspects of Heteroscedasticity
- Unequal Variance: The fundamental characteristic is the inconsistent spread of residuals (the differences between observed and predicted values).
- Impact on Regression: It significantly affects the accuracy and reliability of regression models.
- Detection Techniques: Various statistical tests and graphical methods help identify its presence.
- Mitigation Strategies: Several approaches can be employed to address its adverse effects.
Discussion: Exploring the Connection Between Specific Points and Heteroscedasticity
Subheading: Identifying Heteroscedasticity
Introduction: Correctly identifying heteroscedasticity is the first critical step in addressing its impact. This section explores the various methods used for detection.
Facets:
- Visual Inspection (Residual Plots): Plotting the residuals against the predicted values or independent variables is a straightforward approach. A funnel-shaped pattern or uneven spread suggests heteroscedasticity. For example, if a scatter plot shows residuals increasing in magnitude as the predicted values increase, it’s a strong indicator.
- Formal Statistical Tests: More rigorous methods include the Breusch-Pagan test and the White test. These tests provide a statistical measure of heteroscedasticity, helping to determine if the observed inequality in variance is statistically significant.
- Understanding the Context: Sometimes, the nature of the data itself suggests the potential for heteroscedasticity. For instance, data on income levels often exhibit heteroscedasticity, with greater variance at higher income brackets.
Summary: Combining visual inspection with formal statistical tests offers a robust approach to heteroscedasticity detection. Understanding the data's inherent properties can also provide valuable clues.
Subheading: Types of Heteroscedasticity
Introduction: Heteroscedasticity can manifest in various forms. Understanding these different patterns is crucial for choosing appropriate mitigation strategies.
Facets:
- Linear Heteroscedasticity: The variance of the error term increases or decreases linearly with the independent variable(s). This is a common pattern often visible in residual plots as a clear funnel shape.
- Quadratic Heteroscedasticity: The variance changes non-linearly, often following a quadratic relationship with the independent variable(s). A parabolic shape might be observed in residual plots.
- Clustered Heteroscedasticity: The variance varies across different groups or clusters within the data. This often occurs when different subgroups have distinct variances.
Summary: Different types of heteroscedasticity require tailored approaches for correction. Identifying the specific type helps in choosing the most effective mitigation technique.
Subheading: Consequences of Ignoring Heteroscedasticity
Introduction: Failing to address heteroscedasticity can lead to several significant issues in statistical analysis, compromising the reliability of inferences.
Further Analysis: The primary consequence is the production of inaccurate standard errors. This impacts hypothesis testing, potentially leading to incorrect conclusions about the significance of model parameters. Furthermore, parameter estimates become less efficient, meaning they are not as precise as they could be with homoscedastic data.
Information Table:
Consequence | Explanation | Impact on Analysis |
---|---|---|
Inaccurate Standard Errors | Standard errors are underestimated or overestimated, affecting hypothesis tests. | Type I or Type II errors are more likely. |
Inefficient Estimates | Parameter estimates are less precise and further from the true values. | Reduced confidence in model parameter estimates. |
Biased Hypothesis Tests | The validity of hypothesis tests is compromised. | Inaccurate conclusions about parameter significance. |
Closing: Ignoring heteroscedasticity can significantly undermine the reliability of statistical inferences. Addressing it is crucial for producing trustworthy and valid results.
FAQ: Addressing Common Questions about Heteroscedasticity
Introduction: This section addresses frequently asked questions regarding heteroscedasticity.
Questions:
-
Q: What is the difference between heteroscedasticity and autocorrelation?
A: Heteroscedasticity refers to unequal variance of the error term, while autocorrelation refers to correlation between error terms over time or across observations. -
Q: Can heteroscedasticity be present in time series data? A: Yes, heteroscedasticity can occur in time series data, often manifesting as changing variance over time.
-
Q: How does heteroscedasticity impact confidence intervals? A: Heteroscedasticity can lead to incorrect confidence intervals, making them either too narrow or too wide, thus misrepresenting the uncertainty associated with parameter estimates.
-
Q: Are there any non-parametric methods to deal with heteroscedasticity? A: While many standard approaches are parametric, non-parametric methods like robust regression techniques can be useful in handling heteroscedasticity.
-
Q: What is the role of transformations in mitigating heteroscedasticity? A: Logarithmic or square root transformations of the dependent variable can sometimes stabilize variance and reduce heteroscedasticity.
-
Q: How can I interpret the results of a Breusch-Pagan test? A: A low p-value (typically below a chosen significance level, e.g., 0.05) from a Breusch-Pagan test suggests that heteroscedasticity is present in the data.
Summary: Addressing these common questions clarifies several crucial aspects of heteroscedasticity and its implications for statistical modeling.
Tips for Handling Heteroscedasticity
Introduction: This section provides practical tips for effectively managing heteroscedasticity in your analysis.
Tips:
- Visual Inspection: Always start by visually examining residual plots to get a preliminary sense of the data's variance.
- Formal Testing: Employ formal tests like Breusch-Pagan or White tests to confirm the presence of heteroscedasticity.
- Weighted Least Squares (WLS): If heteroscedasticity is detected, consider applying WLS, which weighs observations based on their variances.
- Data Transformations: Explore transformations of the dependent or independent variables (e.g., logarithmic or square root transformations) to stabilize variance.
- Robust Standard Errors: If transformations are ineffective, consider using robust standard errors, which are less sensitive to heteroscedasticity.
- Consider Alternative Models: In some cases, a different model specification might be more appropriate for the data.
- Expert Consultation: Seek expert advice if you encounter complex or challenging situations.
Summary: A combination of careful data exploration, appropriate statistical tests, and effective mitigation strategies is key to managing heteroscedasticity.
Summary: Understanding and Addressing Heteroscedasticity
This exploration of heteroscedasticity highlights its significant impact on the reliability of statistical analyses. Understanding its different types, detecting its presence, and employing appropriate mitigation strategies are crucial for ensuring the validity and accuracy of statistical inferences. Failing to account for heteroscedasticity can lead to inaccurate conclusions and misguided decision-making based on unreliable model results.
Closing Message: The presence of heteroscedasticity necessitates careful attention and the application of appropriate statistical techniques. By understanding its implications and employing effective mitigation strategies, researchers and analysts can ensure the integrity and robustness of their statistical analyses. Continued research and development in statistical methodology will undoubtedly lead to further advancements in dealing with this pervasive challenge in data analysis.
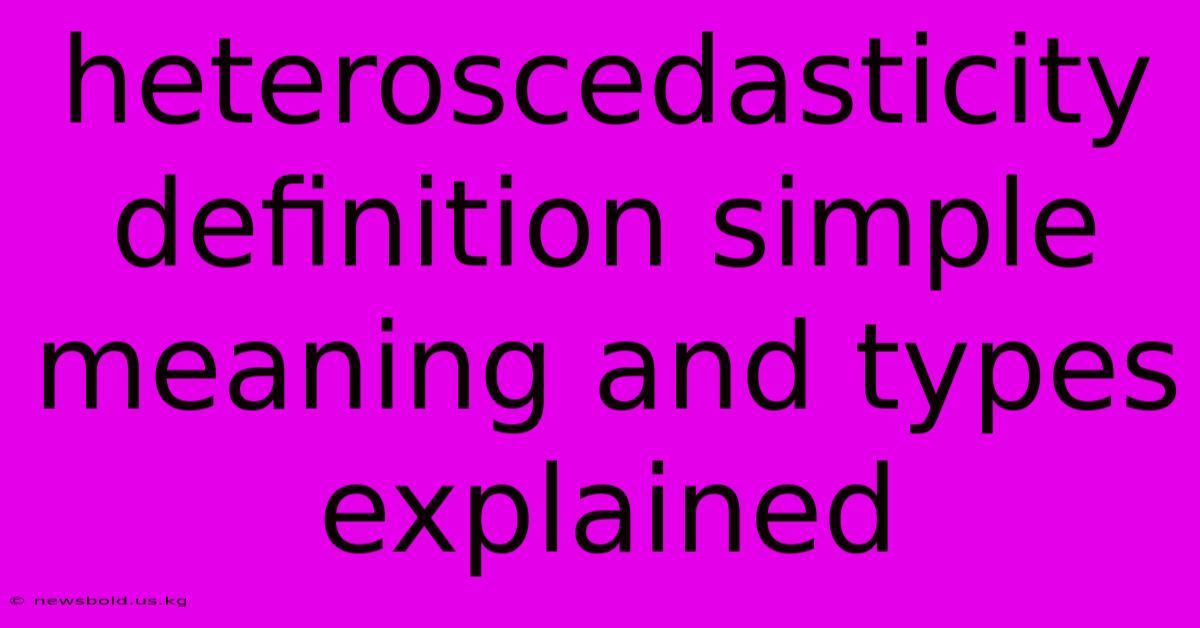
Thank you for taking the time to explore our website Heteroscedasticity Definition Simple Meaning And Types Explained. We hope you find the information useful. Feel free to contact us for any questions, and don’t forget to bookmark us for future visits!
We truly appreciate your visit to explore more about Heteroscedasticity Definition Simple Meaning And Types Explained. Let us know if you need further assistance. Be sure to bookmark this site and visit us again soon!
Featured Posts
-
Good This Month Gtm Definition
Jan 05, 2025
-
Maquliadora Definition History Benefits Worker Explotation
Jan 05, 2025
-
Government Sponsored Enterprise Gse Definition And Examples
Jan 05, 2025
-
Hubbert Curve Definition
Jan 05, 2025
-
Maintenance Margin Definition And Comparison To Margin Accounts
Jan 05, 2025