How Would The Banking Industry Use Business Intelligence
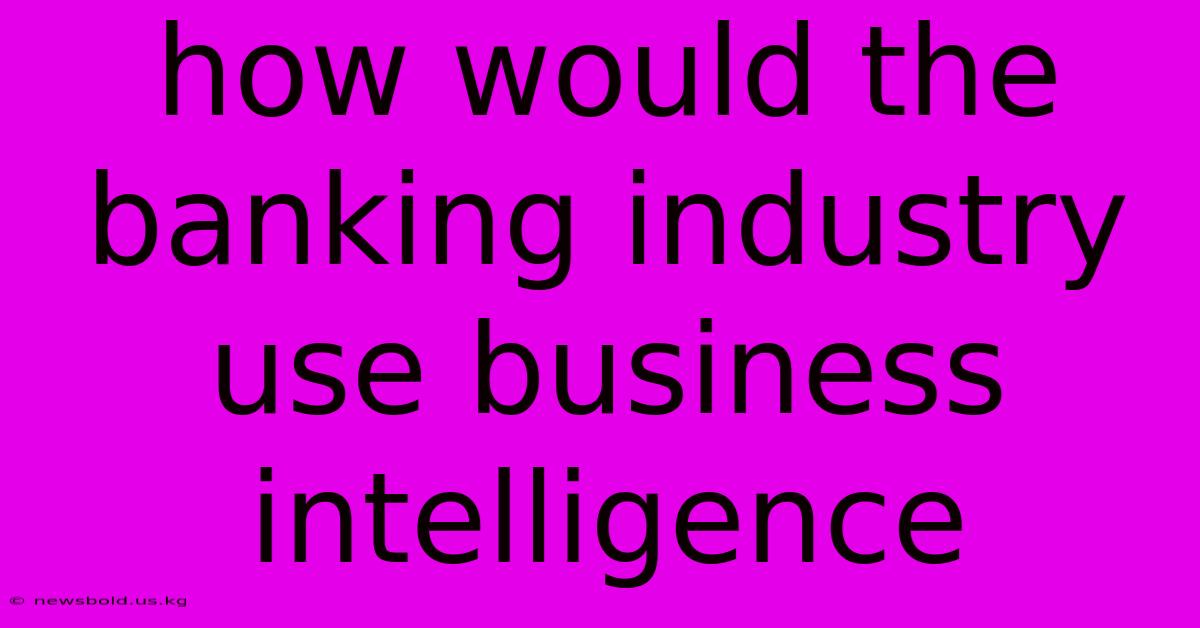
Discover more in-depth information on our site. Click the link below to dive deeper: Visit the Best Website meltwatermedia.ca. Make sure you don’t miss it!
Table of Contents
Unlocking Potential: How Business Intelligence Transforms the Banking Industry
Does the sheer volume of banking data feel overwhelming? It's a goldmine waiting to be explored. Business Intelligence (BI) offers the tools to transform this raw data into actionable insights, driving profitability and enhancing customer experiences. This exploration delves into the multifaceted ways BI reshapes the banking landscape.
Editor's Note: This comprehensive guide on how the banking industry leverages Business Intelligence was published today.
Why It Matters & Summary
The banking industry is data-rich. Transactions, customer interactions, market trends – all generate vast datasets. BI provides the analytical power to sift through this information, revealing patterns and trends otherwise hidden. This translates to improved risk management, enhanced customer service, optimized operations, and ultimately, increased profitability. This article explores key BI applications in banking, from fraud detection and customer segmentation to personalized marketing and regulatory compliance. Keywords include: Business Intelligence, Banking Analytics, Financial Data Analysis, Risk Management, Customer Relationship Management (CRM), Regulatory Compliance, Predictive Modeling, Data Mining, Data Visualization.
Analysis
This analysis draws upon established research in BI applications, case studies of successful banking implementations, and expert opinions from the financial technology sector. The aim is to provide a practical, data-driven overview of how banks are successfully using BI to gain a competitive edge.
Key Takeaways
Application Area | Key Benefits | Example |
---|---|---|
Fraud Detection | Reduced losses, improved security | Real-time anomaly detection, suspicious transaction flagging |
Customer Segmentation | Targeted marketing, personalized offers | Grouping customers by demographics, spending habits, risk profiles |
Risk Management | Improved credit scoring, reduced loan defaults | Predictive modeling of credit risk, early warning systems |
Regulatory Compliance | Streamlined reporting, reduced penalties | Automated reporting, data quality assurance |
Personalized Marketing | Increased customer engagement, improved loyalty | Tailored offers based on individual preferences |
Operational Efficiency | Reduced costs, improved productivity | Optimizing branch locations, automating processes |
Business Intelligence in Banking: A Deep Dive
Introduction: Business Intelligence is no longer a luxury for the banking industry; it's a necessity. Its ability to transform raw data into actionable insights is crucial for competitiveness, profitability, and regulatory compliance. This section outlines key aspects of BI's application within the financial sector.
Key Aspects of BI in Banking:
- Data Integration: Consolidating data from disparate sources (transaction systems, CRM, market data) is fundamental.
- Data Warehousing: Creating a central repository for structured and unstructured data for efficient analysis.
- Data Mining & Analytics: Employing advanced statistical techniques and machine learning algorithms to identify patterns.
- Data Visualization: Presenting complex data in an easily understandable format (dashboards, reports).
- Predictive Modeling: Using historical data to forecast future trends and outcomes (e.g., customer churn, loan defaults).
Discussion: Each of these aspects works synergistically. For instance, effective data integration is the bedrock upon which data warehousing and subsequent analysis are built. Data visualization ensures that the insights gained are readily accessible and usable by decision-makers at all levels.
Data Integration: The Foundation of Success
Introduction: The banking industry's data landscape is incredibly diverse. Data resides in numerous systems, often siloed and incompatible. Effective data integration is paramount for creating a unified view of the customer and the business.
Facets:
- Data Sources: Transaction processing systems, CRM systems, loan origination systems, market data feeds, social media data.
- Integration Techniques: ETL (Extract, Transform, Load) processes, API integrations, data virtualization.
- Challenges: Data inconsistency, data quality issues, security and privacy concerns.
- Mitigations: Data cleansing and standardization, robust security protocols, data governance frameworks.
- Impact: Improved data accuracy, enhanced analytical capabilities, reduced data redundancy.
Summary: Successful data integration lays the groundwork for effective BI implementation. It unlocks a holistic understanding of the customer and business operations, which is essential for making informed decisions.
Predictive Modeling: Forecasting the Future
Introduction: Predictive modeling leverages historical data to forecast future outcomes. In banking, this has significant applications across various domains.
Further Analysis: Predictive models can anticipate customer churn, predict loan defaults, optimize marketing campaigns, and detect fraudulent transactions. These models often employ machine learning algorithms, such as regression analysis, decision trees, or neural networks.
Closing: Accurate predictive modeling empowers banks to proactively manage risks, personalize customer interactions, and optimize their operations for maximum efficiency. However, model accuracy relies heavily on data quality and the appropriate selection of algorithms.
Information Table:
Model Type | Application | Key Metrics | Advantages | Disadvantages |
---|---|---|---|---|
Regression | Credit scoring, risk assessment | R-squared, RMSE | Relatively simple to implement | Assumes linear relationships |
Decision Trees | Customer churn prediction, fraud detection | Accuracy, precision | Easy to interpret, handles non-linear data | Prone to overfitting |
Neural Networks | Fraud detection, market prediction | Accuracy, AUC | High accuracy, handles complex relationships | Difficult to interpret, computationally intensive |
FAQ
Introduction: This section addresses frequently asked questions about Business Intelligence in banking.
Questions:
- Q: What is the ROI of BI in banking? A: The ROI varies depending on implementation, but improvements in efficiency, risk management, and customer satisfaction can significantly outweigh initial investment costs.
- Q: What are the security and privacy concerns related to BI? A: Protecting sensitive customer data is paramount. Robust security measures, including encryption and access controls, are essential.
- Q: How long does it take to implement a BI solution? A: Implementation timelines vary greatly based on the scope and complexity of the project, ranging from months to years.
- Q: What are the key challenges in implementing BI in banking? A: Data integration, data quality, skills gaps, and resistance to change are common challenges.
- Q: What skills are required for successful BI implementation? A: Data scientists, data analysts, data engineers, and business professionals with analytical skills are needed.
- Q: How can banks ensure the accuracy and reliability of their BI insights? A: Robust data governance, quality control processes, and regular model validation are critical.
Summary: Addressing these FAQs provides a clearer understanding of the practical implications and challenges associated with implementing BI in the banking industry.
Tips for Effective BI Implementation in Banking
Introduction: This section offers practical advice for banks seeking to successfully leverage BI.
Tips:
- Start small: Begin with a focused project to demonstrate value before scaling up.
- Prioritize data quality: Invest in data cleansing and standardization.
- Foster collaboration: Ensure that data analysts, IT professionals, and business stakeholders work together.
- Choose the right technology: Select BI tools that meet the bank's specific needs and integrate seamlessly with existing systems.
- Invest in training: Equip staff with the necessary skills to interpret and utilize BI insights.
- Develop a data governance framework: Establish clear policies and procedures for data management and security.
- Measure and monitor: Regularly evaluate the effectiveness of BI initiatives and make adjustments as needed.
Summary: By following these tips, banks can significantly increase their chances of successfully implementing BI and realizing its full potential.
Summary: Business Intelligence offers a transformative opportunity for the banking industry. By harnessing the power of data, banks can improve efficiency, manage risk more effectively, personalize customer experiences, and ultimately achieve greater profitability. The successful implementation of BI requires a strategic approach, a focus on data quality, and a commitment to continuous improvement.
Closing Message: The future of banking is inextricably linked to the effective use of data. Banks that embrace Business Intelligence and leverage its capabilities will be best positioned to thrive in a dynamic and competitive market. Investing in BI is not merely an operational upgrade; it's a strategic imperative for long-term success.
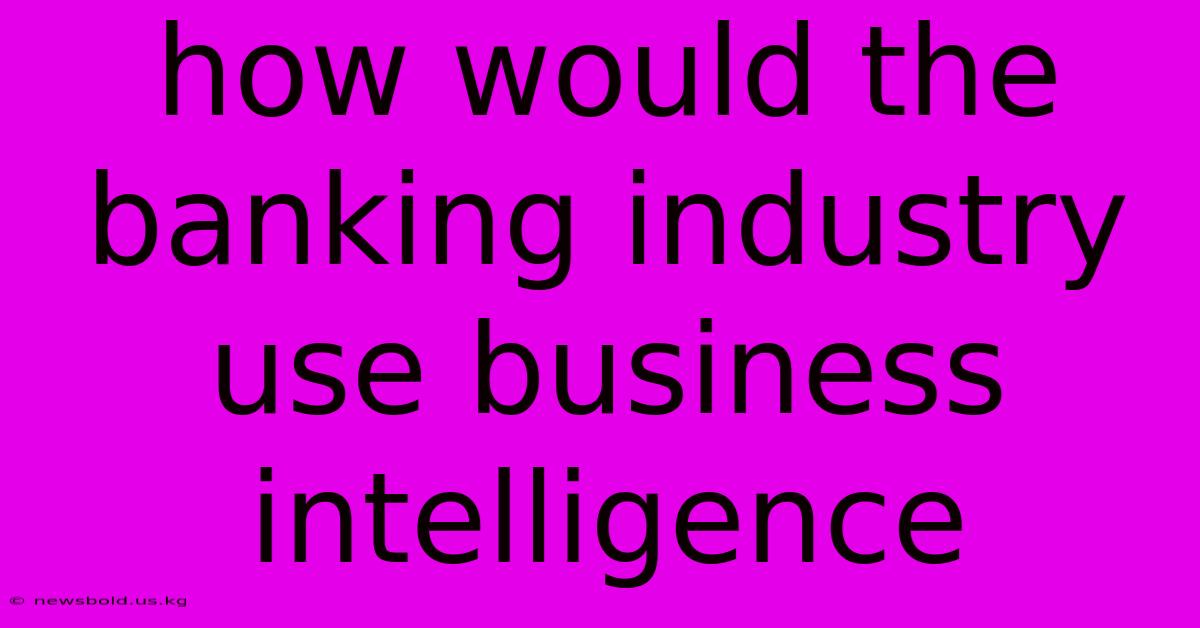
Thank you for taking the time to explore our website How Would The Banking Industry Use Business Intelligence. We hope you find the information useful. Feel free to contact us for any questions, and don’t forget to bookmark us for future visits!
We truly appreciate your visit to explore more about How Would The Banking Industry Use Business Intelligence. Let us know if you need further assistance. Be sure to bookmark this site and visit us again soon!
Featured Posts
-
Present Situation Index Definition
Jan 09, 2025
-
What Credit Bureau Does Paypal Use
Jan 09, 2025
-
Proved Reserves Definition
Jan 09, 2025
-
Which Companies Use Joint Ventures
Jan 09, 2025
-
How To Request Credit Limit Increase Apple
Jan 09, 2025