Hypothesis To Be Tested Definition And 4 Steps For Testing With Example
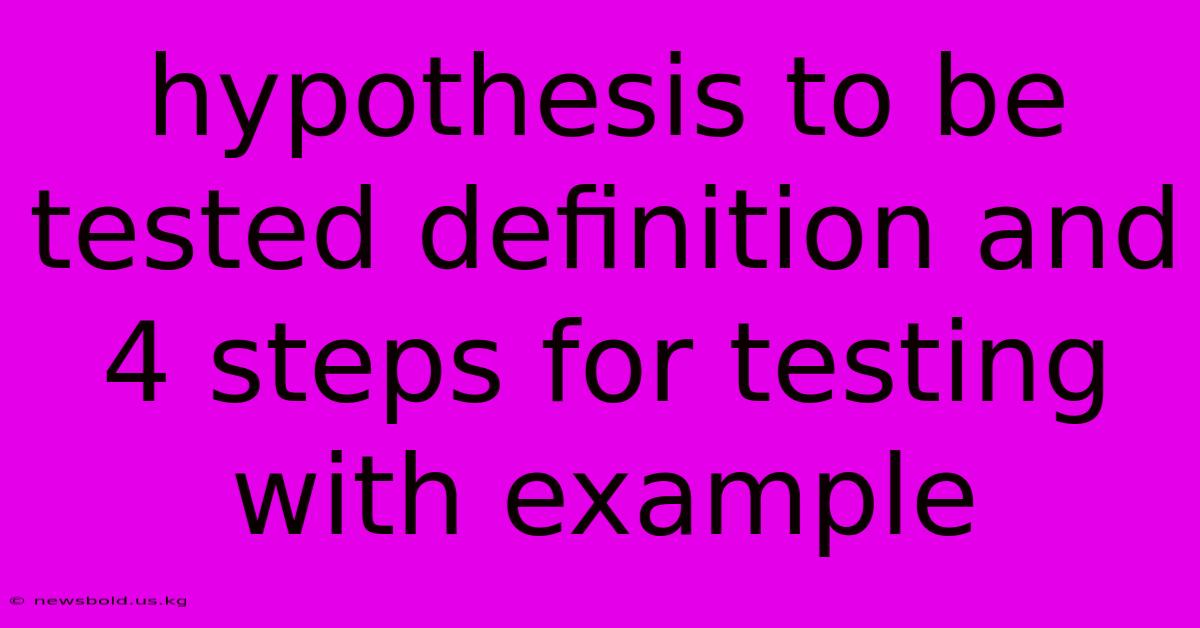
Discover more in-depth information on our site. Click the link below to dive deeper: Visit the Best Website meltwatermedia.ca. Make sure you don’t miss it!
Table of Contents
Unveiling the Hypothesis: Definition and 4 Steps to Test It
What distinguishes a mere hunch from a scientific endeavor? The rigorous process of testing a well-defined hypothesis. This article delves into the precise definition of a testable hypothesis and outlines a four-step process for effective testing, complete with illustrative examples.
Editor's Note: This comprehensive guide to hypothesis testing has been published today, offering a practical framework for researchers and students alike.
Why It Matters & Summary
Understanding and correctly formulating testable hypotheses is crucial for any scientific inquiry or data-driven decision-making process. This article provides a foundational understanding of hypothesis testing, covering its definition, the crucial distinction between testable and untestable hypotheses, and a clear four-step methodology to guide effective testing. The process is explained using relatable examples, emphasizing the importance of clear objectives, data collection, analysis, and interpretation. Keywords covered include: hypothesis testing, null hypothesis, alternative hypothesis, experimental design, data analysis, statistical significance, p-value, scientific method.
Analysis
This guide synthesizes established scientific methodology and statistical principles to provide a practical framework for hypothesis testing. The four-step process described represents a simplified yet effective approach applicable across various fields, from scientific research to business analysis. The examples provided are chosen for their clarity and accessibility, illustrating the core concepts without unnecessary complexity.
Key Takeaways
Point | Description |
---|---|
Hypothesis Definition | A testable statement predicting a relationship between variables. |
Testable vs. Untestable | Differentiates between statements verifiable through observation/experiment and those that are not. |
Four-Step Testing Process | Outlines the stages: formulating hypotheses, designing the experiment, analyzing data, and interpreting results. |
Importance of Replication | Emphasizes the need for repeated testing to confirm findings and build confidence in conclusions. |
Let's transition to a deeper exploration of hypothesis testing.
Hypothesis Testing: A Deep Dive
Introduction
A hypothesis is a specific, testable prediction about the relationship between two or more variables. It forms the cornerstone of scientific investigation, guiding the research process and providing a framework for interpreting results. A hypothesis must be falsifiable; meaning there must be a possible outcome that would disprove it.
Key Aspects
- Null Hypothesis (H₀): This is a statement of "no effect" or "no difference." It proposes that there is no significant relationship between the variables being studied. This is the hypothesis that researchers attempt to reject.
- Alternative Hypothesis (H₁ or Hₐ): This is a statement that contradicts the null hypothesis. It proposes that there is a significant relationship between the variables. This is what researchers hope to support through their investigation.
- Variables: These are the factors that are being measured or manipulated in the experiment. Independent variables are those that are changed or manipulated, while dependent variables are those that are measured and observed.
- Experimental Design: The careful planning and execution of the experiment, including the selection of participants, the control of extraneous variables, and the method of data collection.
- Data Analysis: The application of statistical methods to determine if the observed results are statistically significant.
Discussion
The connection between the clarity of the hypothesis and the successful execution of the experiment is paramount. A poorly defined hypothesis will lead to ambiguous results and difficulties in interpreting the data. For example, a vague statement like "Exercise is good for you" is not a testable hypothesis. A more precise and testable hypothesis might be: "Individuals who engage in 30 minutes of moderate-intensity exercise five times a week will experience a statistically significant decrease in systolic blood pressure compared to a control group."
The Four Steps to Hypothesis Testing
This section outlines a four-step process for rigorously testing a hypothesis.
Step 1: Formulating the Hypotheses
This involves clearly stating both the null and alternative hypotheses. Let's use the exercise example from above.
- Null Hypothesis (H₀): There is no significant difference in systolic blood pressure between individuals who engage in 30 minutes of moderate-intensity exercise five times a week and a control group.
- Alternative Hypothesis (H₁): Individuals who engage in 30 minutes of moderate-intensity exercise five times a week will experience a statistically significant decrease in systolic blood pressure compared to a control group.
Step 2: Designing the Experiment
This involves carefully planning the research methods, participant selection, data collection, and control of extraneous variables. For the exercise example, researchers would need to:
- Recruit participants and randomly assign them to either the exercise group or the control group.
- Define "moderate-intensity exercise" precisely.
- Measure systolic blood pressure at baseline and after a specified period of time.
- Control for other factors that could influence blood pressure, such as diet and smoking habits.
Step 3: Analyzing the Data
Once data is collected, appropriate statistical analysis is performed to determine if the results are statistically significant. This might involve t-tests, ANOVA, or other statistical methods, depending on the type of data and research design. The p-value obtained indicates the probability of obtaining the observed results if the null hypothesis were true. A small p-value (typically less than 0.05) suggests that the null hypothesis can be rejected.
Step 4: Interpreting the Results
Based on the statistical analysis, a conclusion is drawn about whether to reject or fail to reject the null hypothesis. If the p-value is less than 0.05, the null hypothesis is rejected, and the alternative hypothesis is supported. This means that there is sufficient evidence to conclude that the independent variable (exercise) has a statistically significant effect on the dependent variable (systolic blood pressure). If the p-value is greater than 0.05, the null hypothesis is not rejected, indicating insufficient evidence to support the alternative hypothesis. It's important to note that "failing to reject the null hypothesis" does not prove that the null hypothesis is true; it simply means that there is not enough evidence to reject it.
Example: Testing the Effect of Sunlight on Plant Growth
Let's consider another example: Does increased sunlight exposure lead to increased plant growth?
- H₀: Increased sunlight exposure has no significant effect on plant growth.
- H₁: Increased sunlight exposure leads to increased plant growth.
Researchers could design an experiment with two groups of plants: one receiving increased sunlight and a control group receiving normal sunlight. Plant height could be measured regularly over a set period, and statistical analysis (e.g., t-test) could be used to determine if there's a significant difference in growth between the two groups.
FAQ
Introduction: This section addresses frequently asked questions about hypothesis testing.
Questions:
- Q: What is a Type I error? A: A Type I error (false positive) occurs when the null hypothesis is rejected when it is actually true.
- Q: What is a Type II error? A: A Type II error (false negative) occurs when the null hypothesis is not rejected when it is actually false.
- Q: How do I choose the appropriate statistical test? A: The choice of statistical test depends on the type of data (e.g., continuous, categorical) and the research design.
- Q: What is the significance level (alpha)? A: The significance level (alpha) is the probability of making a Type I error (typically set at 0.05).
- Q: What is the power of a test? A: The power of a test is the probability of correctly rejecting the null hypothesis when it is false.
- Q: How important is sample size? A: Adequate sample size is crucial for obtaining reliable and statistically significant results.
Summary: Understanding the nuances of hypothesis testing requires careful consideration of various factors. The selection of appropriate statistical tools and the awareness of potential errors are key to conducting sound research.
Tips for Effective Hypothesis Testing
Introduction: This section offers practical tips to enhance the efficacy of hypothesis testing.
Tips:
- Clearly define variables: Ensure unambiguous definitions for all variables involved.
- Control extraneous variables: Minimize the influence of factors other than the independent variable.
- Use appropriate statistical methods: Select tests tailored to the data type and research design.
- Replicate studies: Repeat the experiment to validate results and enhance confidence.
- Interpret results cautiously: Avoid overgeneralization and acknowledge limitations.
- Consult with statisticians: Seek expert advice for complex designs or analyses.
Summary: Careful planning and execution are crucial for obtaining meaningful and reliable results from hypothesis testing.
Summary
This article provided a comprehensive overview of hypothesis testing, outlining its definition, the importance of formulating testable hypotheses, and a clear four-step process for rigorous testing. The examples and discussion aim to equip readers with a practical understanding of this fundamental scientific process.
Closing Message: Mastering hypothesis testing is a cornerstone of effective scientific inquiry and data-driven decision-making. By applying these principles carefully, researchers can gain valuable insights and contribute meaningfully to their respective fields. Continue to hone your understanding and refine your methods to enhance the quality and impact of your research.
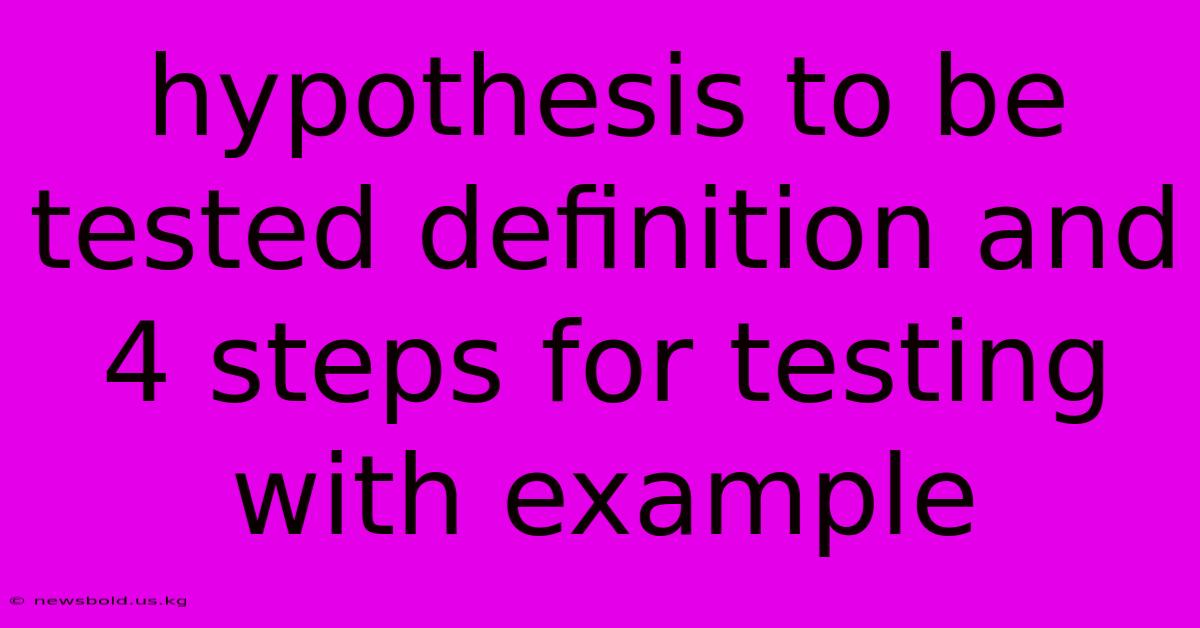
Thank you for taking the time to explore our website Hypothesis To Be Tested Definition And 4 Steps For Testing With Example. We hope you find the information useful. Feel free to contact us for any questions, and don’t forget to bookmark us for future visits!
We truly appreciate your visit to explore more about Hypothesis To Be Tested Definition And 4 Steps For Testing With Example. Let us know if you need further assistance. Be sure to bookmark this site and visit us again soon!
Featured Posts
-
Hollywood Stock Exchange Hsx Definition
Jan 05, 2025
-
Green Investing Definition
Jan 05, 2025
-
In Service Withdrawal Definition Rules Taxes Penalties
Jan 05, 2025
-
What Are The Main Differences Between Forward And Futures Contracts
Jan 05, 2025
-
What Is Fraud Definition Types And Consequences
Jan 05, 2025