Information Coefficient Ic Definition Example And Formula
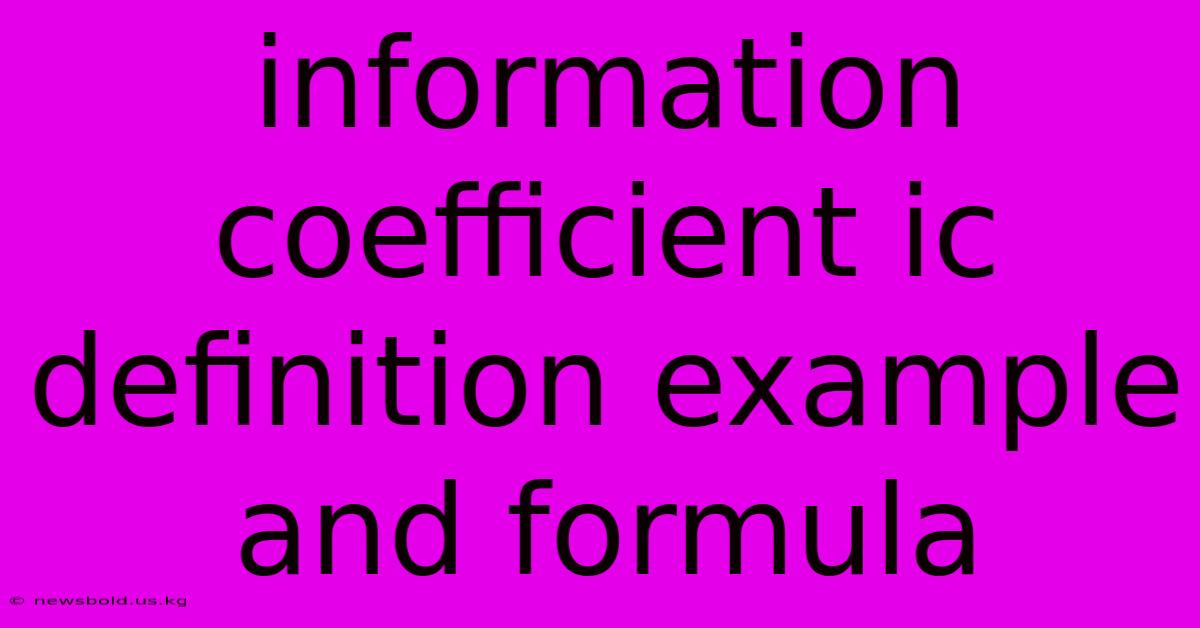
Discover more in-depth information on our site. Click the link below to dive deeper: Visit the Best Website meltwatermedia.ca. Make sure you don’t miss it!
Table of Contents
Unveiling the Information Coefficient: Definition, Formula, and Practical Applications
Does the predictive power of a variable truly reflect its potential in investment strategies? The Information Coefficient (IC) offers a crucial metric for gauging this predictive ability. This comprehensive guide delves into the definition, formula, and practical applications of the IC, providing invaluable insights for informed decision-making.
Editor's Note: This detailed exploration of the Information Coefficient (IC) has been published today.
Why It Matters & Summary
Understanding the Information Coefficient is paramount for investors, analysts, and anyone involved in quantitative finance. The IC quantifies the strength of the relationship between a predictor variable and its target, providing a standardized measure of predictive accuracy irrespective of the scale of the predictor. This summary covers the IC definition, its calculation formula, practical examples, and the critical role it plays in evaluating predictive models, particularly in portfolio management and stock selection. Related terms like correlation, rank correlation, and prediction accuracy are also explored to give a comprehensive understanding.
Analysis
The analysis presented here relies on established statistical principles and practical applications within the finance industry. Real-world examples are used to illustrate the calculation and interpretation of the IC, focusing on how it can help assess the efficacy of various predictive factors and inform investment decisions. The focus remains on clear explanations and practical applications rather than complex mathematical derivations.
Key Takeaways
Aspect | Description |
---|---|
Definition | Measures the correlation between a predictor variable and its future outcome. |
Formula | Spearman Rank Correlation between predicted and actual outcomes |
Interpretation | Ranges from -1 to +1, with higher values indicating stronger predictive power. 0 implies no predictive ability. |
Applications | Evaluating factor models, stock selection, portfolio optimization |
Limitations | Sensitive to data quality, can be affected by data noise and outliers. |
Let's now delve into the specifics.
Information Coefficient (IC)
The Information Coefficient (IC) is a widely used metric in quantitative finance to assess the predictive power of a given variable or factor. It measures the correlation between a predictor variable's rank and the actual rank of the future outcome. The key feature distinguishing IC from simple correlation is its use of ranks, making it less sensitive to outliers and the specific distribution of the predictor variable. This robustness is crucial when dealing with financial data that often exhibits non-normality and significant outliers.
Key Aspects of the Information Coefficient
-
Focus on Rank: The IC utilizes the ranks of both the predictor variable and the outcome, not their raw values. This is critical for reducing the influence of extreme values and making the measure more reliable across different datasets and scales.
-
Spearman's Rank Correlation: The IC is essentially calculated using Spearman's rank correlation coefficient. This method provides a robust measure of monotonic association between two variables, even if the relationship isn't strictly linear.
-
Standardized Measure: The IC value always falls between -1 and +1, making it easily comparable across different predictors and models. A value close to 1 indicates a strong positive predictive ability, a value close to -1 indicates a strong negative predictive ability, and a value near 0 indicates a lack of predictive ability.
The Information Coefficient Formula
The formula for the Information Coefficient utilizes Spearman's rank correlation:
IC = ρ = 1 - (6 * Σd²)/(n(n²-1))
Where:
- ρ (rho): Represents Spearman's rank correlation coefficient, which is equivalent to the IC.
- Σd²: The sum of the squared differences between the ranks of the predictor variable and the ranks of the outcome variable.
- n: The number of observations.
While the formula appears straightforward, calculating it manually can be tedious, especially with large datasets. Statistical software packages readily provide functions for calculating Spearman's rank correlation, making the process efficient and accurate.
Practical Examples of Information Coefficient Calculation
Let's consider a simple example. Suppose we're evaluating a stock selection model that uses "earnings growth" as a predictor variable for future stock returns. We have data for 5 stocks:
Stock | Earnings Growth Rank | Return Rank |
---|---|---|
A | 1 | 2 |
B | 2 | 1 |
C | 3 | 3 |
D | 4 | 4 |
E | 5 | 5 |
- Calculate the difference (d) between the ranks:
Stock | Earnings Growth Rank | Return Rank | d (Difference) | d² (Squared Difference) |
---|---|---|---|---|
A | 1 | 2 | -1 | 1 |
B | 2 | 1 | 1 | 1 |
C | 3 | 3 | 0 | 0 |
D | 4 | 4 | 0 | 0 |
E | 5 | 5 | 0 | 0 |
-
Sum the squared differences (Σd²): Σd² = 1 + 1 + 0 + 0 + 0 = 2
-
Apply the formula:
IC = 1 - (6 * 2) / (5 * (5² - 1)) = 1 - (12) / (5 * 24) = 1 - 12/120 = 1 - 0.1 = 0.9
In this simplified example, the IC is 0.9, indicating a very strong positive correlation between earnings growth rank and future return rank. This suggests that the model using earnings growth as a predictor is quite effective.
Interpreting the Information Coefficient
The interpretation of the IC is straightforward:
-
IC close to +1: Strong positive relationship; higher predictor values are strongly associated with higher outcomes.
-
IC close to -1: Strong negative relationship; higher predictor values are strongly associated with lower outcomes.
-
IC close to 0: Weak or no relationship; the predictor has little or no predictive power.
It's crucial to note that a high IC does not necessarily guarantee perfect predictions. It simply reflects the strength of the statistical association between the predictor and the outcome. Other factors could influence the actual outcome.
Applications of the Information Coefficient
The IC finds widespread applications in various areas of finance:
-
Factor Model Evaluation: Assessing the predictive power of individual factors within a factor model.
-
Stock Selection: Ranking stocks based on their predicted returns using various factors.
-
Portfolio Optimization: Selecting assets with the highest IC values to construct a well-diversified portfolio.
-
Risk Management: Identifying factors that can predict future risk levels.
Limitations of the Information Coefficient
While the IC is a powerful tool, it has limitations:
-
Data Quality: The IC is sensitive to the quality of the underlying data. Inaccurate or incomplete data can lead to misleading results.
-
Overfitting: The IC can be overfitted to historical data, leading to poor out-of-sample performance. Robustness checks and cross-validation are essential.
-
Non-Linear Relationships: While Spearman's rank correlation handles monotonic relationships well, it may not capture complex, non-monotonic relationships adequately.
FAQ
Introduction: This section addresses common questions regarding the Information Coefficient.
Questions and Answers:
-
Q: What is the difference between IC and correlation? A: While both measure association, IC uses ranks, making it robust to outliers and insensitive to the scale of the predictor. Correlation uses raw values.
-
Q: Can IC be negative? A: Yes, a negative IC indicates a negative relationship between the predictor and the outcome.
-
Q: What constitutes a "good" IC value? A: This depends on the context. In some applications, an IC above 0.1 might be considered good, while in others, a much higher value might be required.
-
Q: How does IC help in portfolio management? A: By identifying factors with high ICs, investors can select assets expected to generate superior returns.
-
Q: How does one account for data noise when using IC? A: Careful data cleaning, using robust statistical methods, and cross-validation are crucial to mitigate the impact of noise.
-
Q: Is IC applicable to all asset classes? A: Yes, although the specific predictors and their effectiveness might vary across asset classes.
Summary: The IC is a crucial tool, but its interpretation should always be cautious and context-dependent.
Tips for Utilizing the Information Coefficient Effectively
Introduction: This section offers practical tips for maximizing the effectiveness of the Information Coefficient in predictive modeling.
Tips:
-
Data Cleaning: Thoroughly cleanse your data to remove outliers and errors before calculating the IC.
-
Feature Selection: Select relevant predictor variables carefully to avoid noise and improve predictive accuracy.
-
Cross-Validation: Use techniques like k-fold cross-validation to assess the IC's robustness and avoid overfitting.
-
Statistical Significance: Test for the statistical significance of the IC to ensure the observed relationship is not due to random chance.
-
Contextual Interpretation: Always consider the context of your application when interpreting IC values.
-
Combine with Other Metrics: Use the IC in conjunction with other evaluation metrics like Sharpe ratio or maximum drawdown to gain a holistic view.
-
Regular Monitoring: Regularly reassess the predictive power of factors and update your models to adapt to changing market conditions.
Summary: By carefully applying these tips, you can leverage the Information Coefficient to enhance the effectiveness of your quantitative strategies.
Summary of Information Coefficient Analysis
This exploration of the Information Coefficient has provided a detailed understanding of its definition, formula, interpretation, and practical applications. The IC, calculated using Spearman's rank correlation, offers a robust and standardized measure of a predictor's predictive power, making it an invaluable tool for investment analysis and portfolio management. Understanding its strengths and limitations allows for more informed and effective utilization of this key metric.
Closing Message: The Information Coefficient is a cornerstone in quantitative finance, offering a robust way to evaluate predictive models. By carefully applying this methodology and acknowledging its limitations, researchers and practitioners can enhance their ability to build superior investment strategies and gain a competitive edge in the markets. Continuous refinement of predictive models, using IC as a guide, will remain a vital aspect of success in dynamic financial markets.
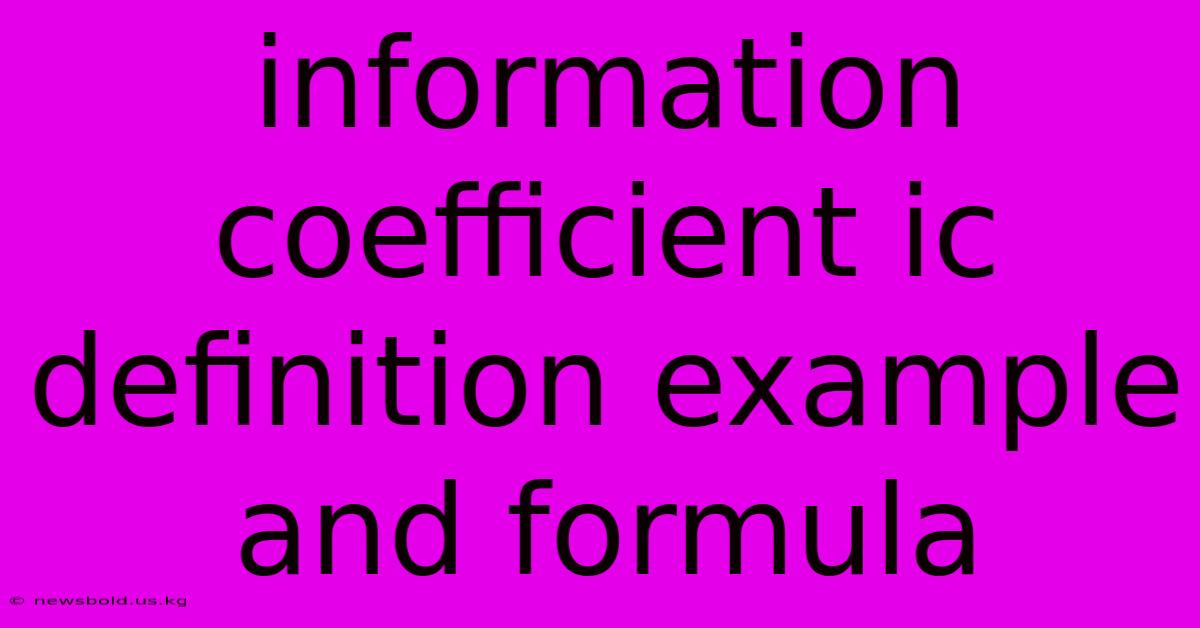
Thank you for taking the time to explore our website Information Coefficient Ic Definition Example And Formula. We hope you find the information useful. Feel free to contact us for any questions, and don’t forget to bookmark us for future visits!
We truly appreciate your visit to explore more about Information Coefficient Ic Definition Example And Formula. Let us know if you need further assistance. Be sure to bookmark this site and visit us again soon!
Featured Posts
-
How To Get Funding To Flip A House
Jan 05, 2025
-
What Is Cash Balance Pension Plan
Jan 05, 2025
-
Hashgraph Consensus Definition
Jan 05, 2025
-
Implicit Rental Rate Definition
Jan 05, 2025
-
Gift Splitting Definition Example And Tax Rules
Jan 05, 2025