Model Risk Definition Management And Examples
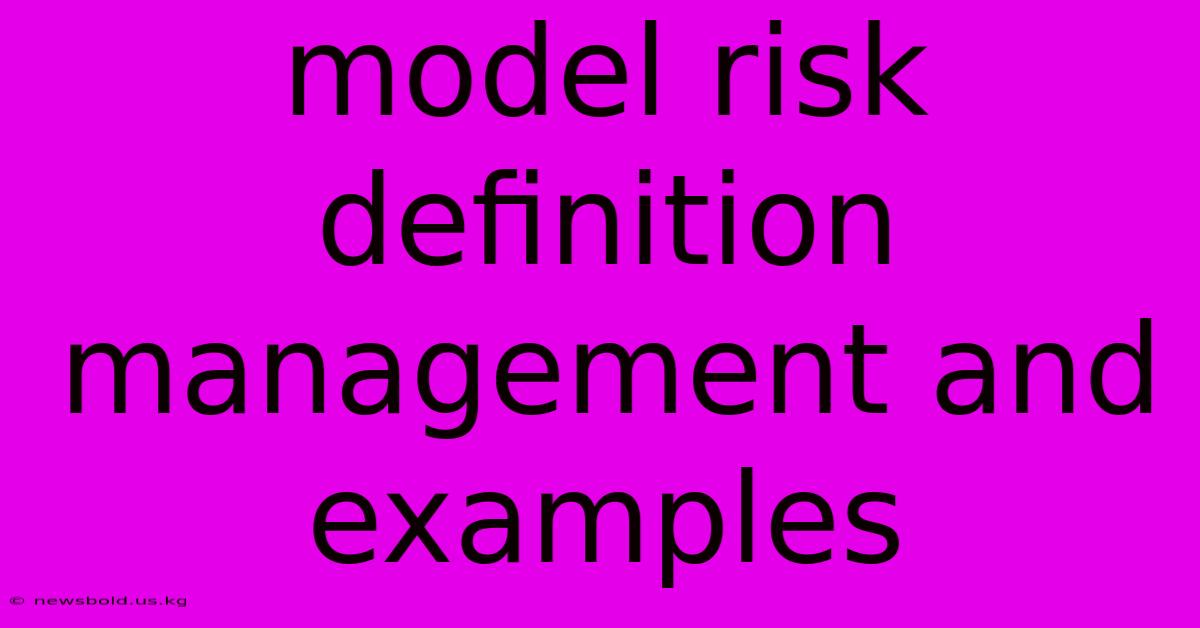
Discover more in-depth information on our site. Click the link below to dive deeper: Visit the Best Website meltwatermedia.ca. Make sure you don’t miss it!
Table of Contents
Unveiling Model Risk: Definition, Management, and Real-World Examples
What defines the potential for significant financial losses stemming from the flaws or misuse of models within financial institutions? The answer lies in understanding model risk. Its management is crucial for maintaining financial stability and protecting against unforeseen catastrophes.
Editor's Note: This comprehensive guide to Model Risk: Definition, Management, and Examples has been published today.
Why It Matters & Summary
Model risk poses a significant threat to financial institutions of all sizes. This article explores the definition, management, and real-world examples of model risk, offering valuable insights into mitigating potential losses. The discussion includes model validation, governance frameworks, and the implications of inaccurate or inadequate models on financial performance and regulatory compliance. Understanding these aspects is vital for risk managers, financial professionals, and anyone interested in the stability of the financial system. Key semantic keywords and LSIs include: model risk management (MRM), quantitative risk management (QRM), model validation, model governance, financial modeling, operational risk, regulatory compliance, and data quality.
Analysis
This exploration of model risk draws upon established research in financial risk management, regulatory guidelines (such as those from the Basel Committee on Banking Supervision), and case studies of real-world model failures. The analysis provides a framework for understanding and addressing model risk, incorporating practical advice for effective management and mitigation strategies. The goal is to equip readers with the knowledge to make informed decisions about model development, implementation, and oversight.
Key Takeaways
Aspect | Description |
---|---|
Model Risk Definition | The potential for loss stemming from the flaws, limitations, or misuse of models used in financial decision-making. |
Management Strategies | Implementing robust validation, governance, and monitoring procedures. |
Real-World Examples | Case studies illustrating the consequences of inadequate model risk management. |
Regulatory Implications | Compliance with relevant regulations and guidelines related to model risk. |
Data Quality | High-quality data is crucial for accurate model outputs and reliable results. |
Model Validation | Rigorous testing and review processes to ensure model accuracy and reliability. |
Subheading: Model Risk
Introduction: Model risk is an inherent aspect of any quantitative model employed in finance, impacting areas such as credit scoring, pricing derivatives, market risk assessment, and regulatory reporting. It represents the potential for inaccurate, incomplete, or inappropriately applied models to lead to significant financial losses.
Key Aspects:
- Data Quality: The accuracy and completeness of input data significantly influence model outputs. Poor data quality can lead to biased and unreliable results.
- Model Specification: The choice of model methodology and underlying assumptions influence the model's validity and predictive power.
- Model Implementation: Errors during the implementation process can compromise the model's accuracy and reliability.
- Model Use and Interpretation: Misinterpreting model outputs or using them inappropriately can lead to poor decision-making.
- Model Monitoring: Regularly monitoring model performance and making necessary adjustments is crucial for maintaining accuracy and reliability.
Discussion: The interconnectedness of these aspects is crucial. For instance, poor data quality (e.g., missing values, outliers) directly impacts model specification, influencing the model's ability to accurately reflect reality. Similarly, even with a well-specified model, incorrect implementation or flawed interpretation can nullify its effectiveness. Effective model risk management requires a holistic approach addressing all key aspects.
Subheading: Data Quality
Introduction: The foundation of any reliable model lies in the quality of its input data. Data issues, such as inaccuracies, inconsistencies, and incompleteness, can significantly distort model outputs, leading to flawed conclusions and potentially substantial financial losses.
Facets:
- Data Accuracy: The degree to which data reflects reality. Inaccurate data leads to biased and unreliable model results. Example: Using outdated market data to price a derivative.
- Data Completeness: The extent to which all necessary data points are available. Missing data can bias model outputs or prevent accurate predictions. Example: Missing credit history data when scoring a loan application.
- Data Consistency: The uniformity of data formats and structures across different sources. Inconsistencies can hinder accurate model construction and interpretation. Example: Different formats for dates across various databases.
- Risks & Mitigations: Data quality issues increase the risk of model failure and inaccurate predictions. Mitigations involve implementing robust data validation and cleansing procedures, employing data governance frameworks, and using appropriate data imputation techniques.
- Impacts & Implications: Poor data quality can lead to incorrect risk assessments, flawed pricing models, and suboptimal business decisions. It can also lead to regulatory violations and reputational damage.
Subheading: Model Validation
Introduction: Model validation is a critical component of model risk management, ensuring the model accurately reflects the intended purpose and performs as expected. A robust validation process assesses the model's soundness, reliability, and appropriateness for its intended application.
Further Analysis: The validation process typically involves several steps:
- Data Validation: Assessing the quality and appropriateness of the data used to build and test the model.
- Model Methodology Review: Evaluating the chosen model's theoretical underpinnings and appropriateness given the application.
- Backtesting: Comparing the model's historical predictions to actual outcomes to assess its accuracy.
- Stress Testing: Evaluating the model's performance under extreme market conditions to identify potential vulnerabilities.
- Sensitivity Analysis: Determining the impact of changes in input variables on the model's output.
Closing: Effective model validation strengthens confidence in the model's output and enhances the overall effectiveness of model risk management. Failing to undertake a thorough validation process substantially increases the risk of model failure.
Information Table: Model Validation Techniques
Technique | Description | Advantages | Limitations |
---|---|---|---|
Backtesting | Comparing model predictions to actual outcomes. | Simple to understand and implement. | Limited insights into model behavior under unusual conditions. |
Stress Testing | Evaluating model performance under extreme conditions. | Identifies model vulnerabilities. | Requires careful selection of stress scenarios. |
Sensitivity Analysis | Assessing the impact of changes in input variables on model output. | Helps understand model behavior and identify key drivers of output. | Can be computationally intensive for complex models. |
Out-of-Sample Testing | Testing the model on data not used in its development. | Provides an unbiased assessment of model generalization ability. | Requires sufficient out-of-sample data. |
Expert Review | Independent assessment of the model by subject matter experts. | Leverages expert knowledge and insights. | Can be subjective and dependent on expertise. |
Subheading: FAQ
Introduction: This section addresses frequently asked questions regarding model risk management.
Questions:
- Q: What are the key regulatory requirements for model risk management? A: Regulatory requirements vary across jurisdictions but often involve robust governance frameworks, comprehensive model documentation, and regular model validation.
- Q: How can data quality issues be addressed? A: Implementing data governance frameworks, establishing clear data quality standards, and employing data cleansing and validation techniques are crucial.
- Q: What are the consequences of inadequate model risk management? A: Inadequate MRM can lead to inaccurate risk assessments, flawed pricing models, regulatory violations, financial losses, and reputational damage.
- Q: How often should models be validated? A: The frequency of model validation depends on several factors, including model complexity, the criticality of its application, and changes in market conditions. Regular reviews are essential.
- Q: What role does senior management play in model risk management? A: Senior management has ultimate responsibility for establishing a strong MRM framework and ensuring its effective implementation and oversight.
- Q: How can organizations cultivate a culture of model risk management? A: Promoting open communication, encouraging independent review, rewarding responsible behavior, and investing in training are vital.
Summary: Addressing these FAQs highlights the multifaceted nature of effective model risk management and the importance of a proactive approach.
Transition: The following section provides practical tips for enhancing model risk management practices.
Subheading: Tips for Effective Model Risk Management
Introduction: This section offers practical strategies for strengthening your organization's approach to model risk management.
Tips:
- Establish a robust governance framework: Define clear roles, responsibilities, and reporting lines for model risk management.
- Implement a comprehensive model inventory: Document all models used within the organization, including their purpose, methodology, and data sources.
- Develop rigorous validation procedures: Ensure models are rigorously tested and validated before deployment.
- Monitor model performance continuously: Regularly monitor model performance and make necessary adjustments to maintain accuracy.
- Invest in skilled personnel: Hire and retain professionals with expertise in model development, validation, and risk management.
- Promote a culture of data integrity: Prioritize data quality, accuracy, and completeness throughout the organization.
- Conduct regular training: Provide ongoing training to staff on model risk management best practices.
- Stay informed of regulatory changes: Keep abreast of any regulatory changes impacting model risk management.
Summary: Implementing these tips significantly improves the effectiveness of model risk management.
Subheading: Summary of Model Risk Management
Summary: This article provided a comprehensive overview of model risk, its definition, management, and real-world examples. Key themes emphasized included the importance of data quality, rigorous model validation, effective governance frameworks, and the consequences of inadequate model risk management.
Closing Message: Effective model risk management is no longer an optional practice but a critical necessity for the stability and success of financial institutions. Proactive approaches to risk mitigation, fostering a culture of responsible model usage, and a deep understanding of regulatory expectations remain crucial factors in navigating the complexities of the financial landscape.
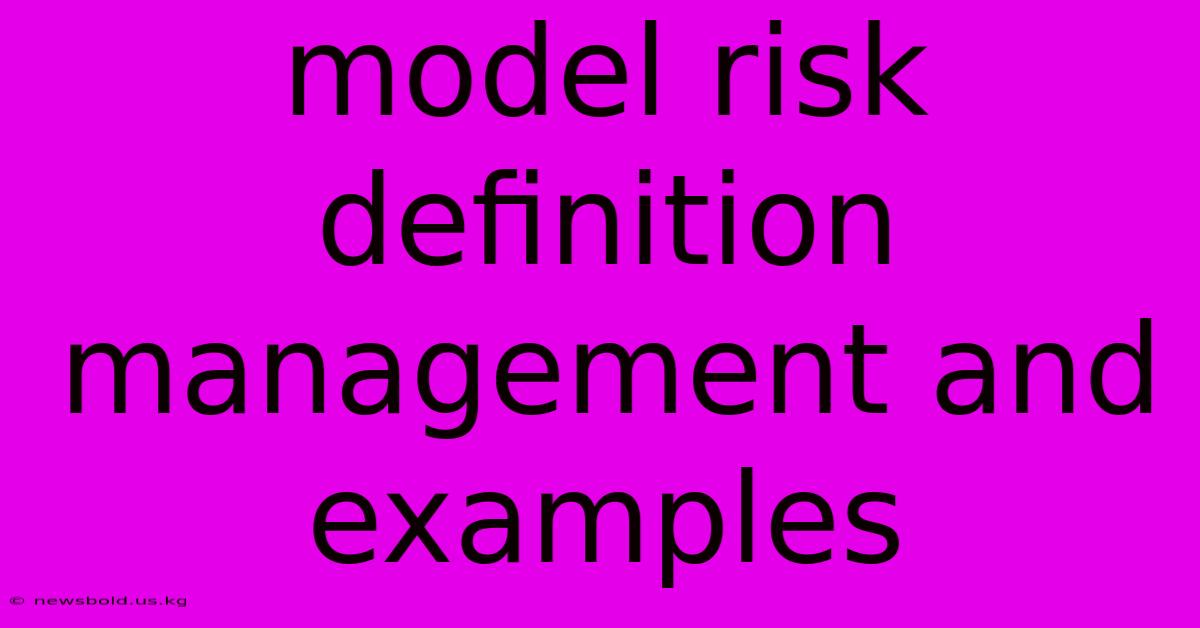
Thank you for taking the time to explore our website Model Risk Definition Management And Examples. We hope you find the information useful. Feel free to contact us for any questions, and don’t forget to bookmark us for future visits!
We truly appreciate your visit to explore more about Model Risk Definition Management And Examples. Let us know if you need further assistance. Be sure to bookmark this site and visit us again soon!
Featured Posts
-
Forward Spread Definition
Jan 05, 2025
-
Where Does Land Go On A Balance Sheet
Jan 05, 2025
-
When Do Crude Futures Contracts Roll
Jan 05, 2025
-
Foreign Bank Supervision Enhancement Act Fbsea Definition
Jan 05, 2025
-
Meander Line Definition
Jan 05, 2025