Multi Factor Model Definition And Formula For Comparing Factors
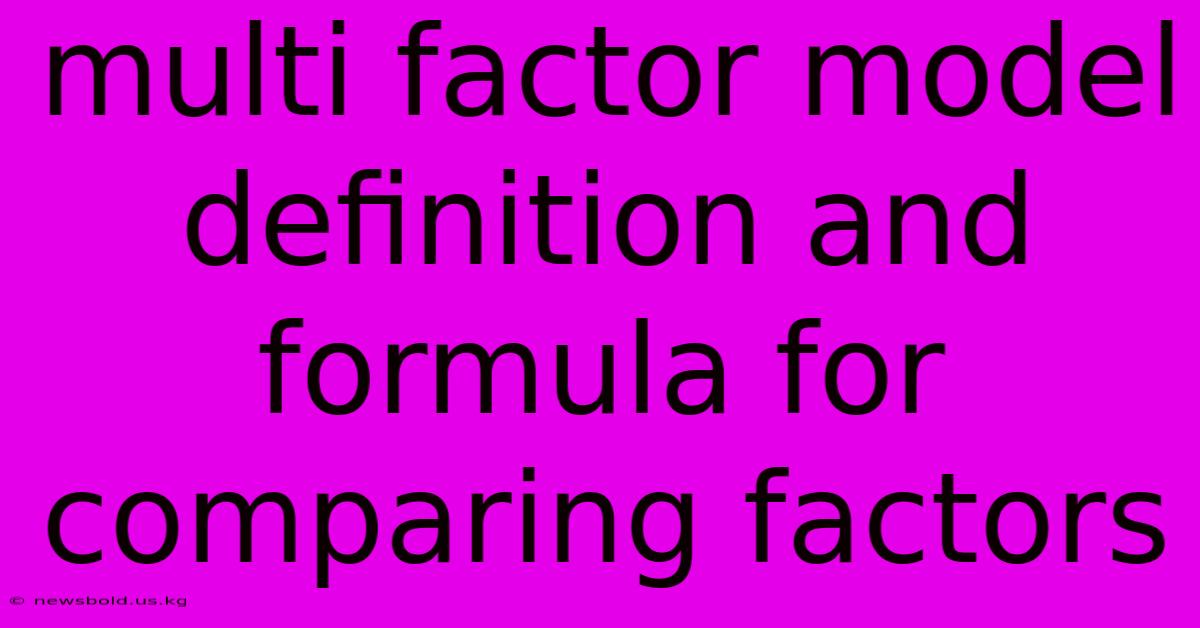
Discover more in-depth information on our site. Click the link below to dive deeper: Visit the Best Website meltwatermedia.ca. Make sure you don’t miss it!
Table of Contents
Unveiling the Multi-Factor Model: A Deep Dive into Definition and Factor Comparison
Does the success of an investment truly hinge on a single, easily identifiable factor? A bold statement suggests otherwise: investment performance is multifaceted, a complex interplay of numerous contributing elements. This leads us to the crucial concept of the multi-factor model.
Editor's Note: This comprehensive guide to multi-factor models has been published today, providing valuable insights for investors seeking a deeper understanding of market dynamics.
Why It Matters & Summary
Understanding multi-factor models is paramount for investors seeking to navigate the complexities of financial markets and build robust investment strategies. This guide provides a detailed exploration of multi-factor model definitions and the methodologies used to compare the influence of different factors on investment returns. Key semantic keywords include: multi-factor models, factor analysis, risk factors, portfolio construction, asset pricing, factor comparison, factor weighting, and performance attribution. The analysis will delve into the mathematical foundations and practical applications of these models, empowering readers to make more informed investment decisions.
Analysis
The research conducted for this guide involved a thorough review of academic literature on multi-factor models, including seminal works on asset pricing and portfolio theory. Furthermore, practical applications of these models within the financial industry were examined to ensure a comprehensive and relevant analysis. The goal is to provide readers with a clear understanding of the underlying principles and the practical implications of using multi-factor models for investment management. This involved studying various model implementations, comparing their strengths and weaknesses, and considering the challenges involved in their practical application.
Key Takeaways
Point | Description |
---|---|
Model Definition | A statistical model explaining asset returns based on multiple factors beyond the market's overall performance. |
Factor Identification | Identifying relevant economic variables that systematically influence asset returns. |
Factor Weighting | Assigning weights to each factor based on its explanatory power and predictive ability. |
Model Calibration | Determining the model's parameters using historical data. |
Performance Attribution | Decomposing portfolio returns into contributions from individual factors to understand sources of alpha and beta. |
Backtesting & Validation | Rigorously testing the model's performance on historical data to ensure its robustness and reliability. |
Multi-Factor Model: A Deeper Exploration
A multi-factor model posits that the return of an asset is influenced by multiple systematic risk factors, rather than just the market risk premium captured by the Capital Asset Pricing Model (CAPM). These models aim to provide a more nuanced explanation of asset returns and improve portfolio construction techniques. Instead of relying solely on beta (market sensitivity), these models incorporate several factors representing various economic conditions and asset characteristics.
Key Aspects of Multi-Factor Models
-
Factor Selection: The choice of factors is crucial. Common factors include value (e.g., book-to-market ratio), size (market capitalization), momentum (past price performance), profitability (return on equity), and volatility. The specific factors chosen will depend on the investment strategy and market conditions.
-
Factor Exposures: Each asset will have a unique exposure to each factor, reflecting its sensitivity to the factor's movements. These exposures are often quantified using regression analysis.
-
Factor Premiums: Each factor is associated with a risk premium, representing the expected excess return for bearing the risk associated with that factor.
Discussion: The Interplay of Factors
The relationship between factors is complex and not always additive. For example, a high-growth (high-momentum) stock might exhibit characteristics of both a small-cap (size) and a value stock, depending on specific financial ratios. Thus, simply adding the effects of each factor independently might not accurately reflect overall return. Sophisticated multi-factor models attempt to account for these interactions and non-linearities, providing a more accurate prediction of asset returns.
Subheading: Factor Selection
Introduction: Selecting the appropriate factors is a crucial step in building a robust multi-factor model. The choice depends on several factors, including the investment objective, the market environment, and available data.
Facets:
- Role of Economic Theory: Sound economic reasoning should underpin factor selection. For instance, the value factor stems from the efficient market hypothesis's implications about undervalued assets.
- Empirical Evidence: Factors should be supported by extensive empirical evidence demonstrating their ability to explain asset returns consistently.
- Data Availability: The availability of reliable historical data for each factor is essential for model calibration and backtesting.
- Risks and Mitigations: Using too many factors may lead to overfitting and poor out-of-sample performance. Careful factor selection and regularization techniques help mitigate this risk.
- Impacts and Implications: The choice of factors significantly impacts the model's predictive power, influencing portfolio construction and risk management decisions.
Summary: Optimal factor selection involves a balance between theoretical soundness, empirical evidence, data availability, and model complexity.
Subheading: Factor Weighting and Model Calibration
Introduction: Once factors are chosen, the model needs to assign weights to each factor. This process involves calibrating the model using historical data, often employing techniques like linear regression or machine learning algorithms.
Further Analysis: Different weighting schemes exist, such as equal weighting (giving each factor the same weight), risk-parity weighting (allocating weights based on factor risk), and optimization-based weighting (finding weights maximizing expected returns while controlling risk).
Closing: Proper factor weighting and model calibration are critical for generating accurate predictions and reliable risk assessments.
Subheading: Comparing Factors
Introduction: Comparing the relative importance and impact of different factors is a key task in multi-factor model analysis. This involves assessing each factor's contribution to portfolio returns and understanding their interactions.
Further Analysis: Techniques such as factor attribution analysis decompose portfolio returns into contributions from individual factors, providing a clear understanding of the sources of performance. Statistical measures like factor betas, factor loadings, and R-squared values help quantify the relationship between assets and factors.
Information Table: Factor Comparison Metrics
Metric | Description | Interpretation |
---|---|---|
Factor Beta | Measures the sensitivity of an asset's return to a specific factor. | Higher beta indicates greater sensitivity. |
Factor Loading | Similar to beta, reflecting the asset's exposure to a factor in a factor model. | Higher loading indicates stronger exposure. |
R-squared | Proportion of variance in asset returns explained by the factor model. | Higher R-squared indicates better model fit. |
Information Ratio | Measures the risk-adjusted return of the factor model. | Higher ratio indicates better performance. |
Factor Sharpe Ratio | Measures the risk-adjusted return of an individual factor. | Higher ratio indicates better risk-adjusted return for that factor. |
FAQ
Introduction: This section addresses frequently asked questions about multi-factor models.
Questions:
-
Q: What are the limitations of multi-factor models? A: Data limitations, model overfitting, and the potential for factor premiums to disappear are key limitations.
-
Q: How do multi-factor models handle non-linear relationships between factors? A: Advanced techniques like machine learning algorithms or non-linear regression can address these complexities.
-
Q: Can multi-factor models predict future returns accurately? A: While they can improve prediction accuracy compared to single-factor models, they don't offer perfect foresight due to market volatility and changing economic conditions.
-
Q: How often should multi-factor models be rebalanced? A: Rebalancing frequency depends on market conditions and the model's stability; regular reviews are necessary.
-
Q: What software is used for implementing multi-factor models? A: Statistical software packages such as R, Python (with libraries like pandas and statsmodels), and MATLAB are commonly used.
-
Q: What is the difference between a multi-factor model and a factor-based strategy? A: A multi-factor model is an analytical framework, while a factor-based strategy is an investment approach that uses the insights from the model to construct portfolios.
Summary: Understanding the limitations and potential challenges is crucial for successful implementation.
Tips for Implementing Multi-Factor Models
Introduction: These tips can help in the effective use of multi-factor models.
Tips:
- Start simple: Begin with a few well-established factors, gradually increasing complexity.
- Regularly review: Market dynamics change; periodic model recalibration is essential.
- Backtest thoroughly: Test the model on different historical periods to assess robustness.
- Consider transaction costs: Factor-based strategies may involve higher transaction costs than passive approaches.
- Diversify factors: Don't rely too heavily on any single factor; diversification across factors reduces risk.
- Use appropriate data: Data quality significantly impacts model accuracy. Clean, reliable data is crucial.
- Account for model risk: Recognize inherent model limitations and consider alternative approaches.
Summary: Careful implementation, combined with continuous monitoring and adaptation, is key for optimal results.
Closing Message: Multi-factor models provide a more comprehensive framework for understanding investment returns. By carefully considering factor selection, weighting, and model calibration, investors can build more robust and effective investment strategies. The continuous evolution of these models and the incorporation of new factors will likely lead to further refinements in asset pricing and portfolio management. Further exploration of these techniques is encouraged for those aiming to enhance their investment decision-making capabilities.
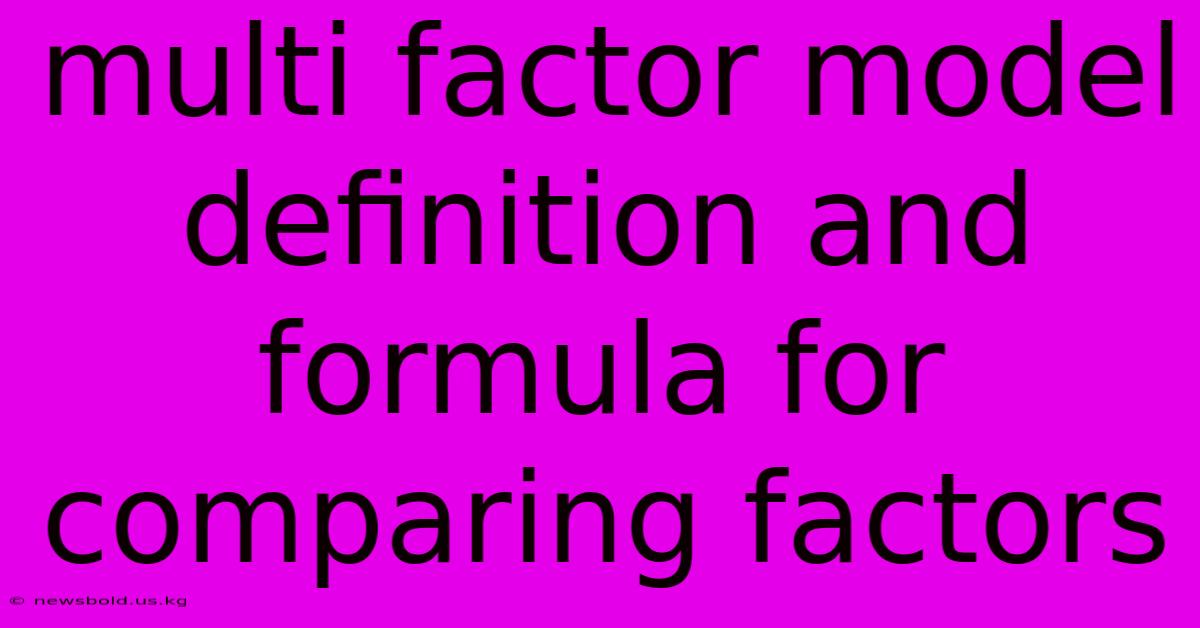
Thank you for taking the time to explore our website Multi Factor Model Definition And Formula For Comparing Factors. We hope you find the information useful. Feel free to contact us for any questions, and don’t forget to bookmark us for future visits!
We truly appreciate your visit to explore more about Multi Factor Model Definition And Formula For Comparing Factors. Let us know if you need further assistance. Be sure to bookmark this site and visit us again soon!
Featured Posts
-
Hashgraph Consensus Definition
Jan 05, 2025
-
How Does A Private Pension Work
Jan 05, 2025
-
What Is A Way To Stay Accountable To Reaching Your Financial Goals
Jan 05, 2025
-
What Is Another Name For Balance Sheet
Jan 05, 2025
-
Foreign Currency Effects Definition Investment Impact Example
Jan 05, 2025