Multiple Discriminant Analysis Mda Definition How Its Used
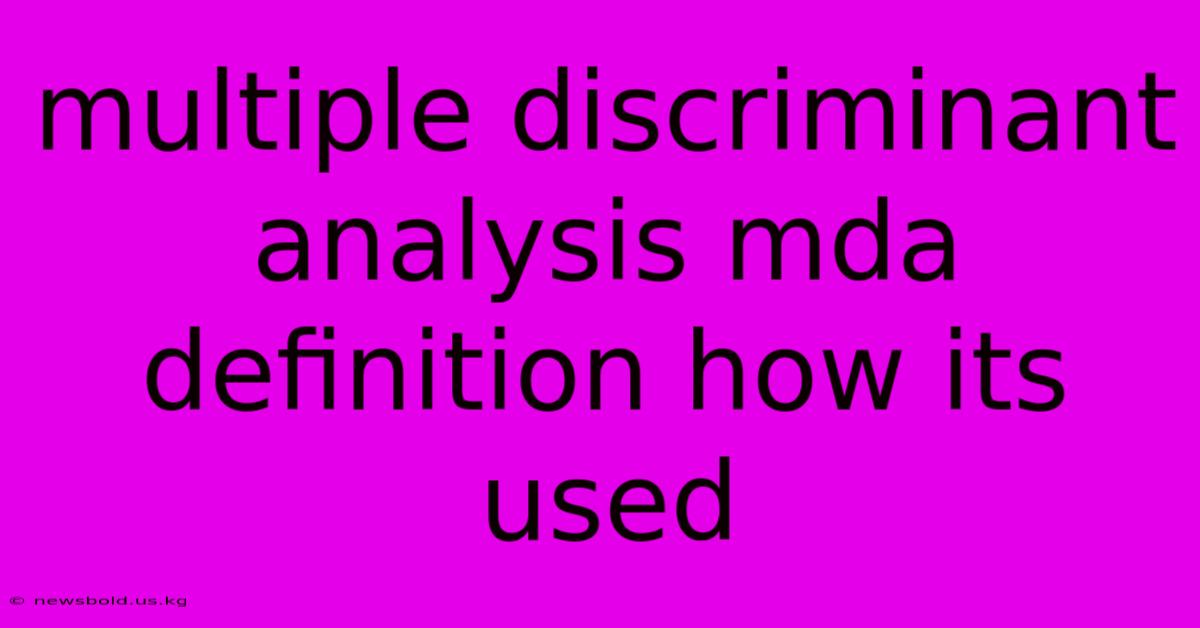
Discover more in-depth information on our site. Click the link below to dive deeper: Visit the Best Website meltwatermedia.ca. Make sure you don’t miss it!
Table of Contents
Unlocking the Power of Multiple Discriminant Analysis (MDA): Definition and Applications
What is Multiple Discriminant Analysis (MDA) and why should we care? MDA is a powerful statistical technique often overlooked, yet crucial for understanding and predicting group membership based on multiple predictor variables. This article will delve into its definition, applications, and significance in various fields.
Editor's Note: This comprehensive guide to Multiple Discriminant Analysis was published today to provide a clear and insightful understanding of this valuable statistical tool.
Why It Matters & Summary: Understanding group differences is paramount across numerous disciplines. MDA offers a sophisticated method to analyze these differences, going beyond simple comparisons. This guide will explore MDA's definition, its underlying principles, its diverse applications (including market segmentation, medical diagnosis, and credit risk assessment), and crucial considerations for its effective implementation. Semantic keywords such as multivariate analysis, classification, prediction, discriminant functions, and canonical correlation will be explored.
Analysis: This analysis draws upon established statistical literature and practical examples to offer a comprehensive understanding of MDA. The methodology involves a systematic review of existing research, focusing on the theoretical underpinnings, practical applications, and limitations of MDA. This structured approach aims to provide a clear, accessible explanation suitable for a broad audience, from students to professionals across diverse fields.
Key Takeaways:
Aspect | Description |
---|---|
Definition | Statistical technique for classifying observations into predefined groups based on multiple predictor variables. |
Purpose | To maximize the separation between groups while minimizing the overlap within groups. |
Output | Discriminant functions, classification functions, and canonical correlation. |
Assumptions | Multivariate normality, homogeneity of variance-covariance matrices, linearity. |
Applications | Market segmentation, medical diagnosis, credit risk assessment, and more. |
Limitations | Sensitivity to outliers, assumptions violation implications. |
Let's transition into a deeper exploration of Multiple Discriminant Analysis.
Multiple Discriminant Analysis (MDA)
Introduction:
Multiple Discriminant Analysis (MDA) is a multivariate statistical technique used to classify individuals or objects into one of several predefined groups based on a set of predictor variables. Unlike simpler methods, MDA simultaneously considers multiple variables to maximize the separation between groups, thereby improving classification accuracy.
Key Aspects:
- Predictor Variables: These are the independent variables used to predict group membership. They can be continuous or categorical.
- Grouping Variable: This is the dependent variable representing the predefined groups. It's a categorical variable with two or more levels.
- Discriminant Functions: These are linear combinations of predictor variables that best discriminate between groups. MDA aims to find the functions that maximize the ratio of between-group variance to within-group variance.
- Classification Function: These are derived from the discriminant functions and used to assign new observations to specific groups.
Discussion:
The core principle of MDA lies in finding linear combinations of the predictor variables (discriminant functions) that maximize the separation between the predefined groups. This separation is quantified by examining the variance between the group means (between-group variance) relative to the variance within each group (within-group variance). The larger the ratio of between-group to within-group variance, the better the separation and the more accurate the classification. The resulting discriminant functions can then be used to classify new observations into the most probable group based on their scores on these functions. The process involves calculating eigenvalues and eigenvectors associated with the discriminant functions, providing insights into the relative importance of each variable in classifying observations. Canonical correlation analysis further aids in quantifying the relationship between the predictor variables and the group variable.
The connection between specific predictor variables and group membership is crucial. For example, in a study classifying customers into high-value and low-value groups, variables like purchase frequency, average order value, and customer lifetime value would be key predictors. MDA will determine how these variables linearly combine to best predict group membership.
Key Predictor Variable: Purchase Frequency
Introduction:
Purchase frequency, a key predictor variable in many MDA applications, specifically reflects the rate at which customers make purchases from a business. Its influence on classification outcomes is significant, reflecting customer loyalty and engagement.
Facets:
- Role: A strong indicator of customer engagement and potential value. High purchase frequency often correlates with higher lifetime value.
- Example: A customer purchasing weekly versus a customer purchasing annually.
- Risks & Mitigations: Over-reliance on frequency alone may misclassify customers with low frequency but high average order value. Combining with other variables mitigates this risk.
- Impacts & Implications: Understanding purchase frequency patterns helps tailor marketing strategies and personalize offers.
Summary:
Purchase frequency's role in MDA is to contribute to the discriminant functions, helping to distinguish high-value from low-value customers. Its inclusion underscores the importance of incorporating multiple facets of customer behavior to achieve accurate classification.
Key Predictor Variable: Average Order Value
Introduction:
Average order value directly relates to the revenue generated by each customer transaction. It provides crucial insight into customer purchasing behavior and spending habits. Understanding its influence within the MDA framework reveals important correlations between the average transaction size and group classification.
Further Analysis:
The average order value can be further analysed to understand its relationship with other variables. For example, the relationship between average order value and the customer's purchase frequency can provide insights into customer segment characteristics. A customer with high purchase frequency and high average order value may represent a lucrative high-value customer. In contrast, a customer with high average order value but low purchase frequency may require different engagement strategies.
Closing:
Average order value is a significant predictor in MDA, assisting in classifying customers based on their overall monetary contribution. By analyzing this variable alongside other factors, a refined understanding of customer behavior and effective segmentation strategies can be achieved.
Information Table: Illustrative data on average order value, purchase frequency, and their contribution to classification (hypothetical data).
Customer Segment | Average Order Value | Purchase Frequency (per month) | Classification (MDA) |
---|---|---|---|
High-Value | $150 | 4 | High-Value |
Medium-Value | $75 | 2 | Medium-Value |
Low-Value | $25 | 1 | Low-Value |
FAQ
Introduction:
This section addresses frequently asked questions about Multiple Discriminant Analysis.
Questions:
-
Q: What are the assumptions of MDA? A: Multivariate normality, homogeneity of variance-covariance matrices, and linearity of relationships between predictors and the grouping variable.
-
Q: How does MDA handle categorical predictor variables? A: They need to be converted into dummy variables (0/1) before applying MDA.
-
Q: What if the assumptions of MDA are violated? A: Robust methods or non-parametric alternatives may be considered.
-
Q: How is the accuracy of MDA assessed? A: Using measures like classification accuracy, sensitivity, specificity, and the confusion matrix.
-
Q: Can MDA be used with a small sample size? A: Ideally, a larger sample size is preferred for reliable results. However, techniques are available to address the challenges of smaller sample sizes.
-
Q: What software packages can perform MDA? A: Most statistical packages such as SPSS, SAS, R, and Python (with libraries like scikit-learn) offer MDA capabilities.
Summary:
Understanding the assumptions and limitations of MDA, along with appropriate evaluation metrics, is critical for effective implementation.
Transition:
Now let's explore some practical tips for using MDA effectively.
Tips for Effective MDA Implementation
Introduction:
These tips aim to guide the effective application of Multiple Discriminant Analysis.
Tips:
- Data Preprocessing: Carefully clean and prepare the data before applying MDA. This includes handling missing values and outliers.
- Variable Selection: Select relevant predictor variables based on theoretical considerations and preliminary analysis. Avoid overfitting.
- Assumption Checking: Verify assumptions of multivariate normality, homogeneity of variance-covariance matrices, and linearity. Consider transformations if necessary.
- Interpretation of Results: Carefully interpret the discriminant functions, understanding the contribution of each predictor variable.
- Cross-Validation: Use cross-validation techniques to assess the generalizability of the MDA model to new data.
- Consider Alternatives: If the assumptions are severely violated, explore alternative multivariate techniques, such as k-nearest neighbors or support vector machines.
Summary:
These tips ensure that the MDA model is appropriately applied, leading to accurate and reliable results.
Summary
This article provided a comprehensive overview of Multiple Discriminant Analysis (MDA), explaining its definition, applications, and essential considerations. The analysis highlighted MDA's importance in various fields, from market research to medical diagnostics. Through examining key predictor variables and addressing frequent questions, this guide provides a practical framework for understanding and implementing this powerful statistical technique.
Closing Message
Multiple Discriminant Analysis represents a valuable tool for understanding group differences and predicting group membership. By carefully considering the assumptions, selecting appropriate variables, and rigorously interpreting the results, researchers and practitioners can leverage MDA's power to make informed decisions across a wide range of applications. Further exploration of advanced MDA techniques and its integration with machine learning algorithms promises even greater insights in the future.
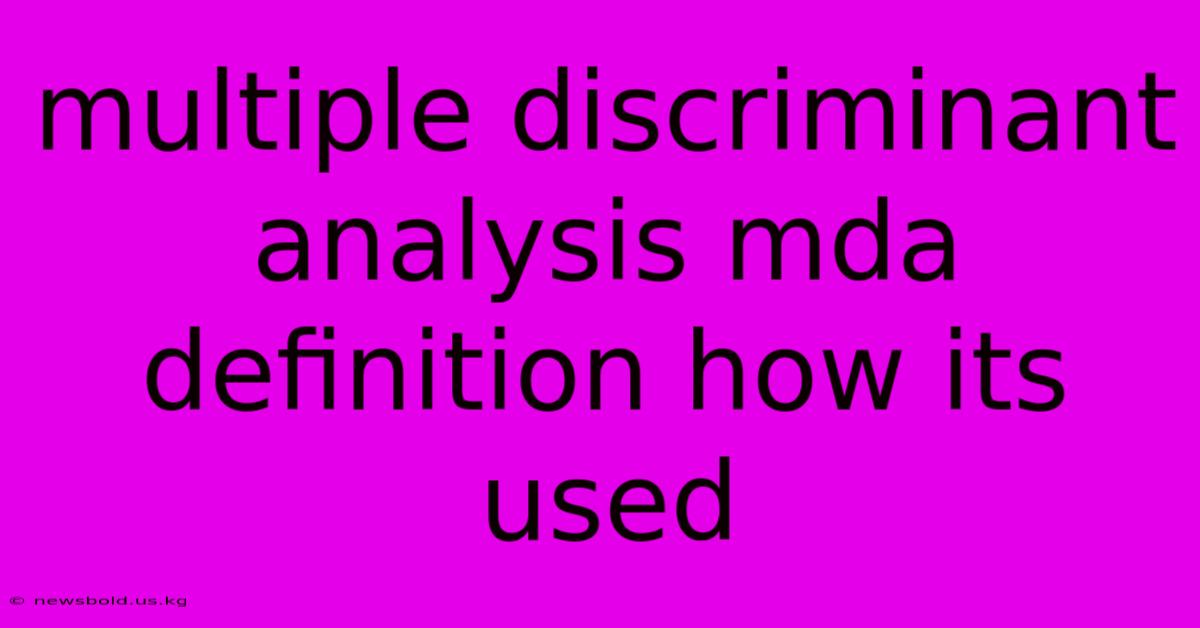
Thank you for taking the time to explore our website Multiple Discriminant Analysis Mda Definition How Its Used. We hope you find the information useful. Feel free to contact us for any questions, and don’t forget to bookmark us for future visits!
We truly appreciate your visit to explore more about Multiple Discriminant Analysis Mda Definition How Its Used. Let us know if you need further assistance. Be sure to bookmark this site and visit us again soon!
Featured Posts
-
Independent Insurance Agents And Brokers Of America Iiaba Definition
Jan 05, 2025
-
Industry Group Definition List Differences With A Market Sector
Jan 05, 2025
-
What Is An Implied Warranty Definition How It Works And Types
Jan 05, 2025
-
Frictional Unemployment Definition Causes And Quit Rate Explained
Jan 05, 2025
-
Macroeconomic Factor Definition Types Examples And Impact
Jan 05, 2025