Recursive Competitive Equilibrium Rce Definition
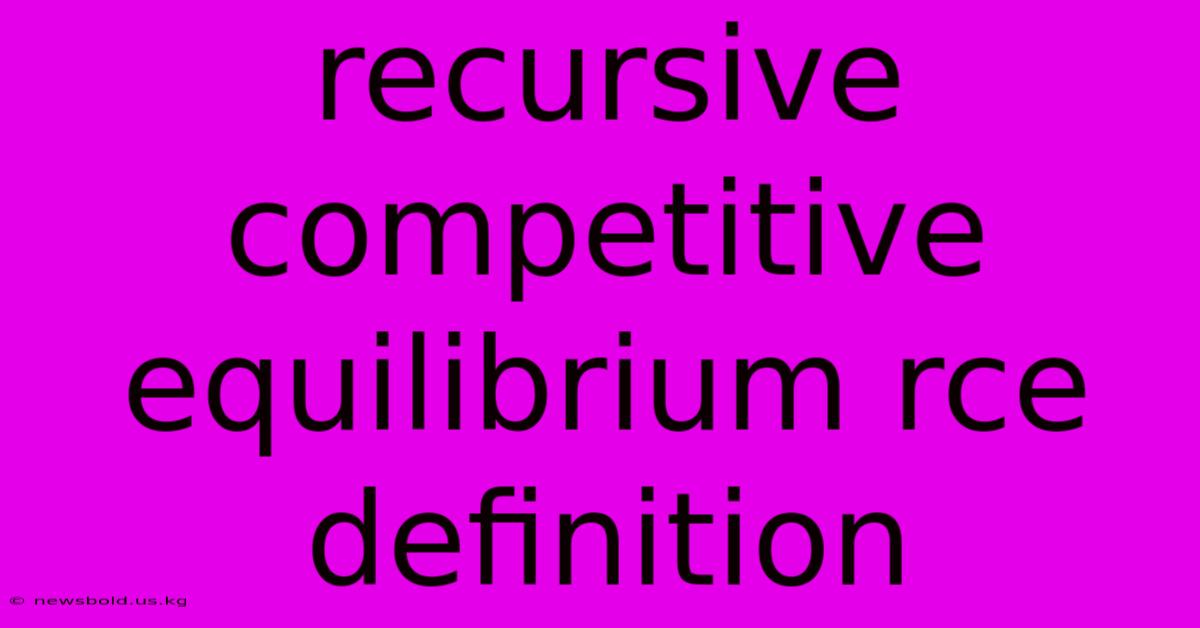
Discover more in-depth information on our site. Click the link below to dive deeper: Visit the Best Website meltwatermedia.ca. Make sure you don’t miss it!
Table of Contents
Unveiling Recursive Competitive Equilibrium (RCE): A Deep Dive into Dynamic Stochastic Economies
What if economic models could accurately capture the complexities of dynamic, uncertain environments? This is precisely the power of Recursive Competitive Equilibrium (RCE), a sophisticated framework offering invaluable insights into long-term economic behavior. This analysis will explore RCE's definition, applications, and implications, revealing its critical role in understanding dynamic stochastic economies.
Editor's Note: This comprehensive guide to Recursive Competitive Equilibrium (RCE) has been published today.
Why It Matters & Summary: Understanding RCE is crucial for economists and policymakers navigating complex real-world scenarios. This framework allows for a rigorous analysis of dynamic decision-making under uncertainty, providing a robust foundation for policy recommendations concerning issues such as macroeconomic stabilization, environmental economics, and asset pricing. The analysis will cover key aspects of RCE, including its definition, underlying assumptions, computational methods, and its applications in various economic fields. Relevant semantic keywords and LSIs include: dynamic stochastic general equilibrium (DSGE), Markov chains, value functions, policy functions, computational economics, rational expectations, and recursive methods.
Analysis: The analysis presented here integrates established economic literature with illustrative examples to provide a clear understanding of RCE. The information is drawn from peer-reviewed journals, reputable textbooks on macroeconomic theory, and computational economics. The goal is to offer a comprehensive guide accessible to advanced undergraduate and graduate students in economics, as well as professionals working in related fields.
Key Takeaways:
Aspect | Description |
---|---|
Definition | A recursive competitive equilibrium is an equilibrium concept in dynamic stochastic economies where agents make optimal decisions based on value functions and policy functions derived from recursive formulations of the economy's dynamic programming problem. |
Key Feature | Recursive structure; breaks down complex intertemporal problems into simpler, sequential ones. |
Assumptions | Rational expectations, perfect competition, complete markets (often relaxed). |
Solution Method | Dynamic programming, numerical methods (e.g., value function iteration). |
Applications | Macroeconomics, asset pricing, environmental economics, resource management. |
Recursive Competitive Equilibrium
Introduction: RCE is a powerful tool for analyzing dynamic stochastic economies, where agents make decisions over time under uncertainty. Unlike static models, RCE explicitly incorporates time and uncertainty, allowing for a more realistic representation of economic phenomena.
Key Aspects:
- Dynamic Programming: This forms the cornerstone of RCE, breaking down the overall intertemporal optimization problem into a sequence of simpler period-by-period problems.
- Value Functions: These functions represent the maximum expected utility an agent can achieve at any point in time, given the current state of the economy and future expectations.
- Policy Functions: These functions describe the optimal actions agents take in each period, given the current state of the economy.
- Rational Expectations: Agents form their expectations about the future based on the true underlying model of the economy. This ensures consistency between agents' forecasts and actual outcomes.
- Market Clearing: The equilibrium condition requires that supply equals demand in each market at each point in time.
Discussion: The key to understanding RCE lies in its recursive structure. By breaking down a complex dynamic problem into a series of simpler, sequential problems, the analysis becomes manageable. This recursive structure allows for the application of dynamic programming techniques to solve for the optimal decisions of agents and the resulting equilibrium. The solution typically involves iteratively solving for value functions and policy functions until convergence is achieved. This process often requires numerical methods due to the complexity of the problem.
The Role of State Variables in RCE
Introduction: The state variables in an RCE model are crucial; they encapsulate all the relevant information from the past that affects current and future decisions. This section elaborates on their significance.
Facets:
- Role: State variables summarize the history of the economy, influencing agent's expectations and choices. Examples include capital stock, technology level, and the distribution of wealth.
- Examples: In a growth model, the capital stock is a key state variable. In an asset pricing model, the level of aggregate consumption may be crucial.
- Risks and Mitigations: Incorrect specification of state variables can lead to inaccurate predictions. Rigorous model specification and sensitivity analysis are crucial mitigations.
- Impacts and Implications: The choice of state variables fundamentally shapes the model's dynamics and predictions. A richer set of state variables can lead to a more realistic, albeit more complex, model.
Summary: The careful selection and inclusion of relevant state variables are paramount in constructing a meaningful and accurate RCE model. Their role in shaping agent expectations and influencing equilibrium outcomes cannot be overstated.
Computational Methods in RCE
Introduction: Solving RCE models frequently requires sophisticated computational methods due to the inherent complexity of dynamic optimization problems. This section will explore some commonly used techniques.
Further Analysis: Value function iteration is a widely used algorithm for solving RCE models. It involves iteratively updating the value function until it converges to a fixed point. Other methods include policy function iteration and projection methods. The specific method chosen often depends on the complexity of the model and the desired level of accuracy.
Closing: The computational burden of solving RCE models can be substantial. Advances in computational power and algorithm design have made it possible to solve increasingly complex models, furthering our ability to analyze dynamic stochastic economies.
Information Table: A comparison of common computational methods for solving RCE models:
Method | Description | Advantages | Disadvantages |
---|---|---|---|
Value Function Iteration | Iteratively updates the value function until convergence. | Relatively straightforward to implement. | Can be slow to converge, especially for high-dimensional problems. |
Policy Function Iteration | Iteratively updates the policy function until convergence. | Can be faster than value function iteration. | Requires careful selection of initial policy function. |
Projection Methods | Approximate the value function using a finite-dimensional basis function. | Can handle high-dimensional problems. | Accuracy depends on the choice of basis functions. |
FAQ
Introduction: This section addresses frequently asked questions about RCE.
Questions:
-
Q: What are the limitations of RCE? A: RCE models can be computationally intensive, and their realism hinges on the accuracy of underlying assumptions, which may not always hold in practice. Oversimplification of agent behavior or market structure is also a common limitation.
-
Q: How does RCE compare to other dynamic equilibrium models? A: RCE offers a more flexible and general framework compared to simpler dynamic models. It incorporates uncertainty explicitly and allows for a rich variety of agent behaviors and market structures.
-
Q: What are some real-world applications of RCE? A: RCE is used extensively in macroeconomics to analyze business cycles, monetary policy, and fiscal policy. It also finds application in asset pricing, environmental economics, and resource management.
-
Q: What is the role of expectations in RCE? A: Rational expectations are central to RCE. Agents' forecasts of future variables must be consistent with the model's true dynamics.
-
Q: How does one choose the appropriate state variables in an RCE model? A: The choice of state variables depends on the specific economic question being addressed. It’s crucial to include all variables that significantly influence agent decisions and market outcomes.
-
Q: Are there alternative solution methods besides dynamic programming? A: While dynamic programming is the most common approach, alternative numerical methods exist, each with trade-offs in terms of computational efficiency and accuracy.
Summary: This FAQ highlights key aspects of RCE, addressing common questions and offering a clearer understanding of this powerful framework.
Tips for Understanding RCE
Introduction: This section offers practical tips for effectively grasping the concepts of RCE.
Tips:
- Start with simpler models: Before tackling complex RCE models, begin with simpler examples to develop a strong foundation in dynamic programming.
- Focus on the intuition: Understanding the underlying economic logic is as important as the mathematical formalism. Visualizations and simulations can be helpful in this regard.
- Utilize computational tools: Familiarize yourself with software packages designed for solving dynamic economic models (e.g., Dynare, Matlab).
- Consult existing literature: Explore published RCE models to see how the framework is applied in various contexts. This can be beneficial in understanding the process.
- Collaborate with others: Working with colleagues or peers can facilitate a deeper understanding of challenging concepts.
Summary: Utilizing these tips can facilitate a better understanding of RCE and its applications.
Summary
This analysis provided a comprehensive exploration of Recursive Competitive Equilibrium, covering its definition, key features, computational methods, and applications. RCE stands as a powerful tool for analyzing dynamic stochastic economies, offering valuable insights into long-term economic behavior.
Closing Message: The continued development and refinement of RCE models promise even deeper insights into complex economic phenomena. Further research in computational methods and model specification will undoubtedly expand the framework’s applicability and enhance our understanding of dynamic economic systems.
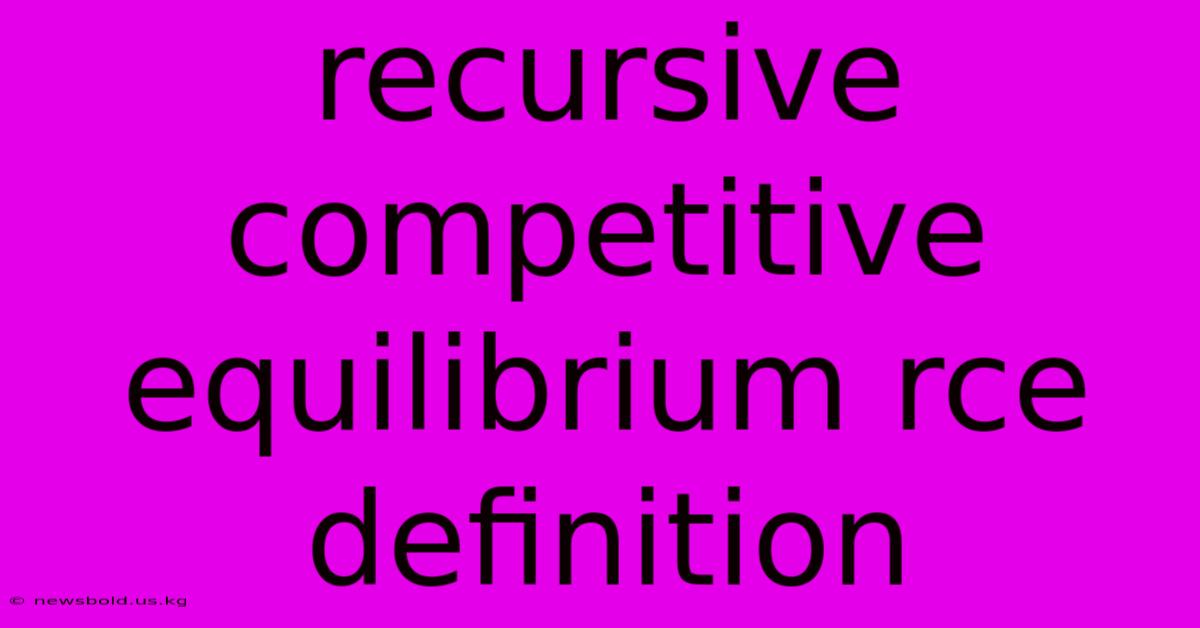
Thank you for taking the time to explore our website Recursive Competitive Equilibrium Rce Definition. We hope you find the information useful. Feel free to contact us for any questions, and don’t forget to bookmark us for future visits!
We truly appreciate your visit to explore more about Recursive Competitive Equilibrium Rce Definition. Let us know if you need further assistance. Be sure to bookmark this site and visit us again soon!
Featured Posts
-
What Is Atm Credit Chase
Jan 09, 2025
-
Where Is The Shenzhen Stock Exchange
Jan 09, 2025
-
Pull Through Production Definition
Jan 09, 2025
-
How To Open Credit Union
Jan 09, 2025
-
What Is Current Balance And Available Credit
Jan 09, 2025