Spurious Correlation Definition How It Works And Examples
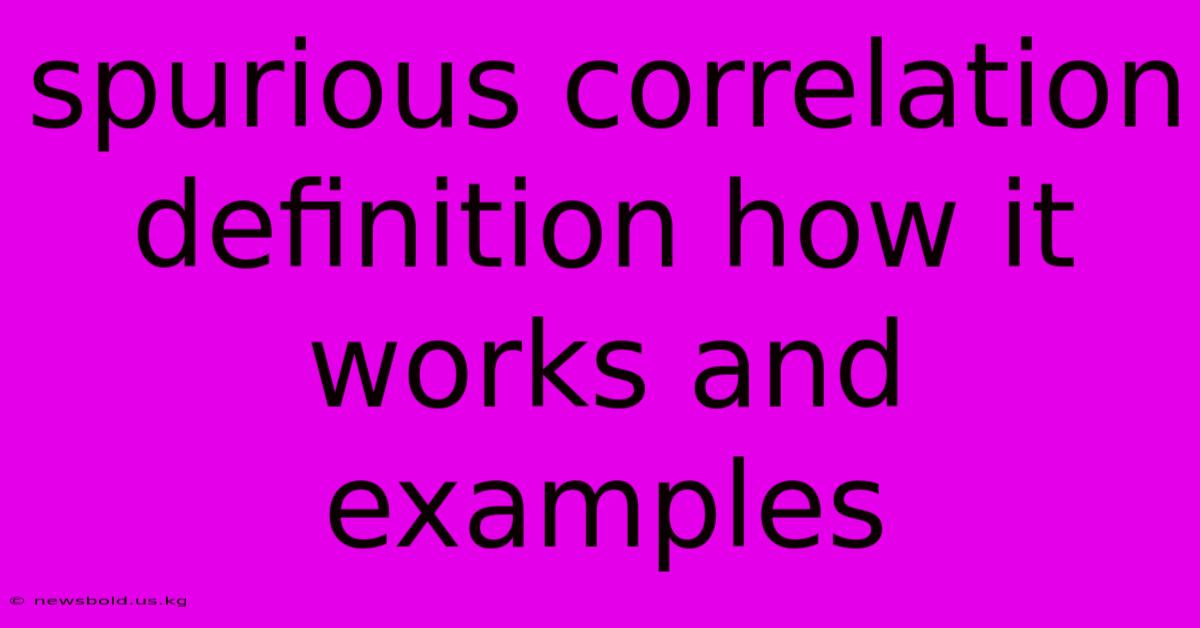
Discover more in-depth information on our site. Click the link below to dive deeper: Visit the Best Website meltwatermedia.ca. Make sure you don’t miss it!
Table of Contents
Unveiling Spurious Correlations: Insights & Examples
What happens when two seemingly unrelated events move in tandem, creating an illusion of causality where none exists? This is the essence of a spurious correlation, a deceptive statistical relationship that can lead to flawed conclusions. This exploration delves into the definition, mechanisms, and illustrative examples of spurious correlations, highlighting their importance in critical thinking and data analysis.
Editor's Note: This article on spurious correlations was published today.
Why It Matters & Summary
Understanding spurious correlations is crucial for anyone interpreting data, from researchers and policymakers to journalists and everyday consumers of information. Misinterpreting these coincidences can lead to misguided policies, flawed predictions, and a general misunderstanding of cause and effect. This article summarizes the definition of spurious correlations, explores how they arise, and presents compelling real-world examples to illustrate their deceptive nature. Keywords include: spurious correlation, correlation, causation, statistical analysis, confounding variables, illusory correlation, misleading statistics, data interpretation.
Analysis
This analysis utilizes a combination of established statistical principles, real-world examples sourced from reputable studies and news reports, and logical reasoning to demonstrate the concept of spurious correlations. The examples are chosen for their clarity and ability to illustrate different mechanisms by which spurious correlations can occur. The aim is to equip readers with the tools to identify and avoid the pitfalls of misinterpreting statistical relationships.
Key Takeaways
Point | Description |
---|---|
Definition | A spurious correlation is a relationship between two or more variables that appears to be causal but is not. |
Mechanisms | Arises from confounding variables, coincidence, or flawed data analysis. |
Identification | Requires careful consideration of potential confounding factors and rigorous statistical analysis. |
Consequences of Misuse | Can lead to incorrect conclusions, flawed policies, and wasted resources. |
Importance of Critical Thinking | Crucial for interpreting data correctly and avoiding misleading conclusions. |
Spurious Correlation: A Closer Look
A spurious correlation describes a relationship between two variables that appears statistically significant but lacks a genuine causal link. The observed correlation is often due to a third, unseen factor, known as a confounding variable, that influences both variables. It's a statistical illusion, where coincidence masquerades as causality.
Key Aspects of Spurious Correlations:
- Lack of Causality: The core characteristic is the absence of a direct causal relationship. One variable doesn't actually cause a change in the other.
- Confounding Variables: A third, often overlooked variable, typically underlies the apparent correlation. This variable independently affects both variables of interest.
- Coincidence: In some cases, a spurious correlation can simply be a matter of random chance, particularly in smaller datasets.
- Data Limitations: Errors in data collection, analysis, or interpretation can also contribute to spurious correlations.
Discussion: Exploring the Mechanisms of Spurious Correlations
Let's delve into the key mechanisms that create these deceptive relationships.
1. Confounding Variables:
This is the most common source of spurious correlations. Imagine a study showing a positive correlation between ice cream sales and drowning incidents. A naïve interpretation might suggest that eating ice cream causes drowning. However, the confounding variable is the summer season. Both ice cream sales and swimming (and thus, drowning risk) increase during warmer months. The correlation is spurious because the summer season drives both variables, not a causal link between ice cream and drowning.
2. Coincidence:
Sometimes, correlations arise purely by chance. With a large number of variables, random fluctuations can create seemingly strong correlations that are meaningless. Consider a scenario where the price of avocados correlates with the number of pirates. This is highly unlikely to represent a causal link; it is simply a coincidence.
3. Data Limitations and Errors:
Poor data quality can also lead to false correlations. Data errors, measurement biases, or selection bias (choosing a non-representative sample) can distort the relationships between variables, leading to misleading results.
2. Confounding Variables: A Deeper Dive
Let's examine the role of confounding variables in detail. Their presence can dramatically distort the observed relationship between two variables.
Facets of Confounding Variables:
- Role: Acts as an unseen driver, influencing both observed variables independently.
- Examples: Seasonality (as in the ice cream/drowning example), socioeconomic factors, age, geographical location.
- Risks and Mitigations: Failing to account for confounding variables leads to inaccurate conclusions. Mitigations include statistical controls (e.g., regression analysis) and careful study design.
- Impacts and Implications: Misinterpreting spurious correlations due to confounding variables can have serious consequences, leading to flawed policies and wasted resources.
Summary: Understanding and addressing confounding variables is essential for accurately interpreting data and avoiding the trap of spurious correlations. Careful study design, rigorous statistical analysis, and awareness of potential confounding factors are crucial steps in preventing this.
3. Coincidence: The Role of Chance
This section explores how pure coincidence can generate spurious correlations, especially in situations with large datasets or many variables being analyzed.
Further Analysis:
The more variables examined, the higher the probability of finding spurious correlations due to chance. This highlights the importance of considering the context and conducting appropriate statistical tests to determine significance. Statistical significance alone isn't sufficient; the correlation must also be meaningful and plausible within the context of the variables involved.
Closing: While chance can lead to spurious correlations, careful statistical analysis and critical thinking can help distinguish between meaningful relationships and mere coincidences.
Information Table: Examples of Spurious Correlations
Correlation | Apparent Causal Link | True Explanation | Confounding Variable(s) |
---|---|---|---|
Ice cream sales & Drownings | Ice cream causes drowning | Both increase in warmer months | Season (summer) |
Shoe size & Reading ability | Larger feet = better reader | Age is the confounding factor | Age |
Number of firefighters & Fire damage | More firefighters = more damage | Larger fires require more firefighters | Fire size |
Nicolas Cage movie releases & Pool drownings | More NC movies = more drownings | Pure coincidence | None (pure chance) |
FAQ
Introduction: This section addresses frequently asked questions about spurious correlations.
Questions:
-
Q: How can I tell if a correlation is spurious? A: Careful examination of potential confounding variables, rigorous statistical analysis (controlling for confounders), and consideration of the plausibility of a causal link are needed.
-
Q: What are the dangers of misinterpreting spurious correlations? A: Misguided policies, wasted resources, and an overall misunderstanding of cause-and-effect relationships.
-
Q: Are all correlations causal? A: No, correlation does not imply causation. Spurious correlations demonstrate this clearly.
-
Q: How common are spurious correlations? A: They can be surprisingly common, especially when dealing with large datasets and many variables.
-
Q: What statistical methods are used to address spurious correlations? A: Regression analysis, controlling for confounding variables, and other multivariate statistical techniques.
-
Q: Can machine learning algorithms identify spurious correlations? A: Machine learning can identify correlations, but interpreting them requires careful human analysis to determine causality and rule out spuriousness.
Summary: Understanding spurious correlations is key to responsible data analysis and interpretation.
Tips for Avoiding Spurious Correlations
Introduction: These tips aim to equip readers with strategies for identifying and avoiding the pitfalls of spurious correlations.
Tips:
-
Consider Confounding Variables: Always explore potential third variables influencing your data.
-
Visualize Your Data: Scatter plots and other visualizations can help spot unusual relationships.
-
Use Robust Statistical Methods: Employ techniques that account for potential confounders.
-
Replicate Your Findings: Independent studies help verify results and rule out chance findings.
-
Be Skeptical: Question the plausibility of any observed correlation before drawing conclusions.
-
Consult Experts: Seek guidance from statisticians or domain experts for complex analyses.
-
Look for Plausible Mechanisms: Is there a plausible biological, social, or physical process that links the variables?
Summary: Following these tips will increase the accuracy and reliability of your interpretations.
Summary
This exploration of spurious correlations has highlighted their deceptive nature and the importance of critical thinking in data analysis. Understanding the mechanisms that create these misleading relationships – confounding variables, coincidence, and data limitations – is essential for drawing accurate conclusions.
Closing Message: The ability to distinguish true causal relationships from mere coincidences is a vital skill for anyone working with data. By practicing critical thinking and utilizing appropriate statistical techniques, the pitfalls of spurious correlations can be avoided, leading to more accurate understanding and informed decision-making.
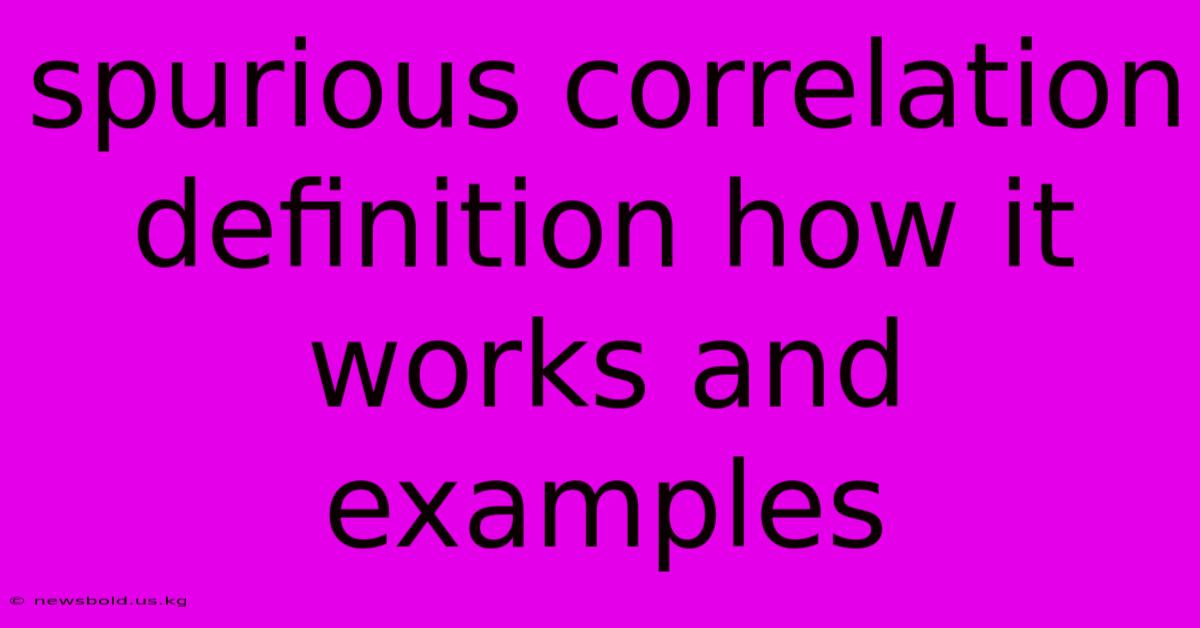
Thank you for taking the time to explore our website Spurious Correlation Definition How It Works And Examples. We hope you find the information useful. Feel free to contact us for any questions, and don’t forget to bookmark us for future visits!
We truly appreciate your visit to explore more about Spurious Correlation Definition How It Works And Examples. Let us know if you need further assistance. Be sure to bookmark this site and visit us again soon!
Featured Posts
-
Tax Liability Definition Calculation And Example
Jan 08, 2025
-
Statement Shock Definition
Jan 08, 2025
-
Authorization Date Definition
Jan 08, 2025
-
Slow Market Definition
Jan 08, 2025
-
Average Daily Trading Volume Adtv Definition How To Use It
Jan 08, 2025