Survival Analysis Definition
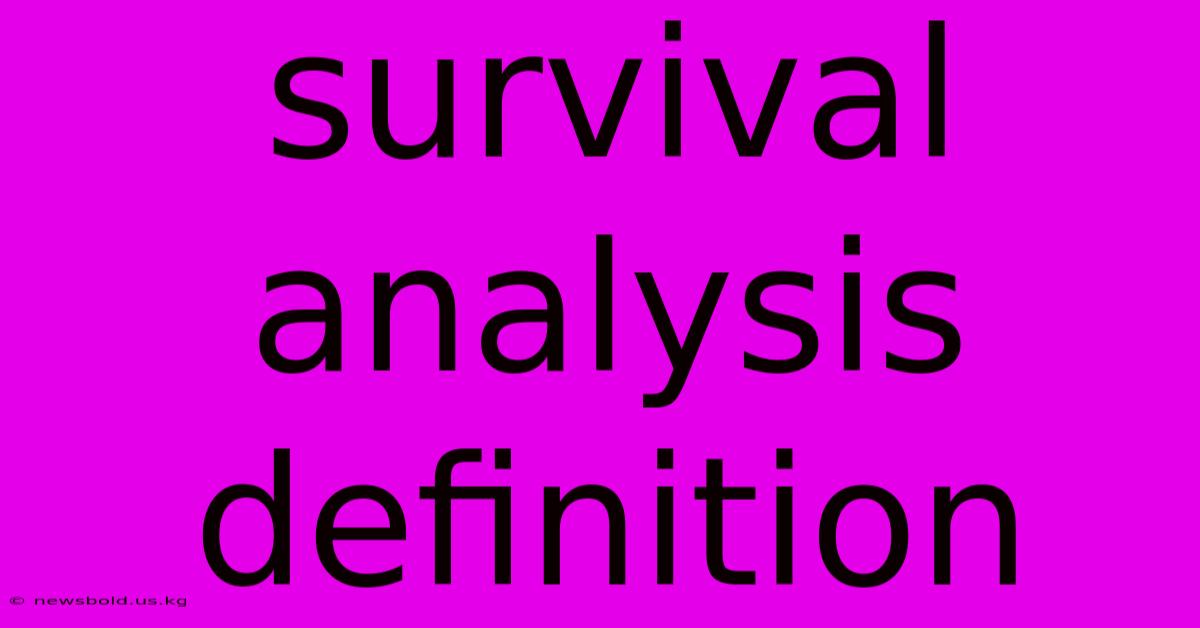
Discover more in-depth information on our site. Click the link below to dive deeper: Visit the Best Website meltwatermedia.ca. Make sure you don’t miss it!
Table of Contents
Unveiling the Secrets of Survival Analysis: A Comprehensive Guide
Hook: Have you ever wondered how researchers predict the lifespan of medical devices, the longevity of customer relationships, or the lifespan of a machine? The answer lies in a powerful statistical technique called survival analysis. This field offers crucial insights into the duration of time until an event of interest occurs, impacting numerous fields from healthcare to engineering.
Editor's Note: This comprehensive guide to survival analysis has been published today.
Why It Matters & Summary: Understanding survival analysis is crucial for making informed decisions in various sectors. This statistical method allows researchers to model and analyze time-to-event data, providing valuable predictions and insights into phenomena where the time until a specific event is the primary focus. This guide will explore the fundamental concepts, techniques, and applications of survival analysis, using clear examples and explanations. Key concepts covered include censoring, hazard functions, Kaplan-Meier curves, and Cox proportional hazards models. The analysis will focus on applications across diverse fields, highlighting the importance and benefits of this powerful statistical tool.
Analysis: This guide provides a comprehensive overview of survival analysis by synthesizing information from reputable statistical textbooks, academic journals, and online resources. The information presented is grounded in established statistical principles and aims to facilitate a clear understanding of the core concepts and methodologies. Examples are drawn from diverse fields to illustrate the broad applicability of survival analysis.
Key Takeaways:
Point | Description |
---|---|
Time-to-Event Data | Data focusing on the time until a specific event occurs. |
Censoring | Handling incomplete data where the event of interest hasn't occurred by the end of the observation period. |
Hazard Function | The instantaneous risk of the event occurring at a specific time, given survival up to that time. |
Kaplan-Meier Estimator | A non-parametric method for estimating the survival function. |
Cox Proportional Hazards Model | A semi-parametric method for modeling the relationship between covariates and survival time. |
Subheading: Survival Analysis
Introduction: Survival analysis is a branch of statistics focusing on analyzing the time until an event occurs. Unlike traditional statistical methods that primarily analyze the mean or average of a variable, survival analysis considers the time-to-event data and handles the complexities introduced by censoring.
Key Aspects:
- Time-to-Event Data: The core of survival analysis revolves around observing the time until a particular event happens. This could be anything from the time until a patient experiences a relapse, the lifespan of a machine part, or the duration of a customer subscription.
- Censoring: A unique feature of survival analysis is its ability to handle censored data. Censoring occurs when the event of interest is not observed within the study period. For instance, a patient might be alive at the end of a clinical trial, or a machine part may still be functioning at the end of the observation period. This incomplete information needs to be accounted for in the analysis. Different types of censoring exist (right, left, and interval).
- Survival Function: This function describes the probability of surviving beyond a specific time point. It's a decreasing function, starting at 1 (everyone is alive at time zero) and approaching 0 as time goes on.
- Hazard Function: This function describes the instantaneous risk of an event occurring at a specific time, given that the individual has survived up to that point. It’s crucial in understanding the risk profile over time.
Discussion:
The key to understanding survival analysis lies in grasping the concept of censoring. Consider a clinical trial studying the effectiveness of a new drug for a specific disease. Some patients might experience remission (the event of interest) during the trial, while others might not. The trial concludes before these latter patients experience remission; their survival times are censored. Ignoring these censored observations would bias the results.
Subheading: Censoring
Introduction: Censoring is inherent to most time-to-event studies. It significantly impacts how the data is analyzed, as ignoring it can lead to inaccurate and misleading conclusions.
Facets:
- Right Censoring: This is the most common type. The event of interest has not occurred by the end of the study. For example, a patient might still be alive at the end of a clinical trial.
- Left Censoring: The event occurred before the start of the observation period. For example, a patient might have already developed a disease before enrolling in a study.
- Interval Censoring: The event occurred within a known interval. For instance, a patient's disease might have been detected sometime between two check-ups.
- Roles: Correctly handling censoring is crucial for obtaining unbiased estimates of survival probabilities and hazard rates. Appropriate statistical methods account for the presence of censored observations.
- Examples: In a manufacturing setting, right-censoring occurs if a machine component is still functioning at the end of the observation period. In an epidemiological study, left-censoring can arise if a patient's disease onset occurred before the study began.
- Risks and Mitigations: Failure to properly account for censoring can lead to biased estimates and unreliable conclusions. Careful study design and the use of appropriate statistical methods are vital to mitigate this risk.
- Impacts and Implications: The presence of censoring influences the choice of statistical methods used in survival analysis. Techniques like the Kaplan-Meier estimator and Cox proportional hazards models are specifically designed to handle censored data.
Summary: Understanding the different types of censoring and their impact on analysis is essential for conducting valid survival analyses. The choice of statistical technique should be guided by the nature and extent of censoring present in the data.
Subheading: Kaplan-Meier Estimator
Introduction: The Kaplan-Meier estimator is a non-parametric method that provides an estimate of the survival function. It directly addresses censoring.
Further Analysis: The Kaplan-Meier curve visually represents the survival function, showing the probability of surviving beyond a specific time point. This curve is widely used to compare survival experiences between different groups. For instance, one might compare the survival curves of patients receiving a new treatment versus a standard treatment.
Closing: The Kaplan-Meier estimator is a fundamental tool in survival analysis, providing a robust and intuitive way to estimate and visualize survival probabilities in the presence of censoring. It is particularly valuable when assumptions of parametric models are not met.
Information Table: Comparison of Kaplan-Meier and Cox Proportional Hazards Model
Feature | Kaplan-Meier | Cox Proportional Hazards Model |
---|---|---|
Type | Non-parametric | Semi-parametric |
Assumptions | Few assumptions | Assumes proportional hazards |
Output | Survival curve, estimated survival probabilities | Hazard ratios, regression coefficients |
Use Cases | Comparing survival curves between groups | Modeling the effect of covariates on survival |
Handling of Covariates | Does not directly model covariates | Directly models the effects of covariates |
Subheading: FAQ
Introduction: This section addresses frequently asked questions about survival analysis.
Questions:
-
Q: What is the difference between a survival function and a hazard function? A: The survival function gives the probability of surviving beyond a given time, while the hazard function gives the instantaneous risk of an event at a given time, given survival up to that time.
-
Q: How does censoring affect the analysis? A: Censoring introduces incomplete information, requiring special statistical methods to avoid bias in estimations of survival probabilities.
-
Q: What is a Cox proportional hazards model? A: It's a regression model used to analyze the effect of multiple covariates on the hazard rate, assuming proportional hazards.
-
Q: Can survival analysis be used with discrete or continuous time data? A: Both; the methods adapt depending on the nature of the time-to-event variable.
-
Q: What are some software packages used for survival analysis? A: R, SAS, SPSS, and Stata all provide extensive tools for survival analysis.
-
Q: What are some common applications of survival analysis beyond medicine? A: It's widely used in engineering (reliability analysis), marketing (customer churn), and finance (credit risk).
Summary: These FAQs highlight key aspects of survival analysis and its diverse applications.
Subheading: Tips for Understanding Survival Analysis
Introduction: Mastering survival analysis requires careful attention to key concepts and proper interpretation of results.
Tips:
- Start with the basics: Understand the core concepts of time-to-event data, censoring, and the survival function before delving into more advanced techniques.
- Visualize the data: Use Kaplan-Meier curves to visually represent survival probabilities and compare groups.
- Understand the assumptions: Parametric models have specific assumptions. Check if these assumptions are met before applying them.
- Choose the right method: Select an appropriate method based on the nature of your data and research question.
- Interpret the results carefully: Focus on the clinical or practical implications of the findings, not just the statistical significance.
- Consider potential biases: Be aware of potential biases related to censoring and study design.
Summary: By following these tips, researchers can enhance their understanding and application of survival analysis methods.
Summary: This guide provided a comprehensive introduction to survival analysis, covering key concepts, methods, and applications. The discussion highlighted the importance of handling censoring correctly and selecting the appropriate statistical technique for analyzing time-to-event data.
Closing Message: Survival analysis is a versatile statistical technique with broad applications across many fields. By understanding its fundamental principles and methods, researchers can gain valuable insights into the duration of time until an event of interest occurs, ultimately leading to better decision-making and improved outcomes. Further exploration of advanced techniques and software packages is recommended for a deeper understanding of this powerful statistical tool.
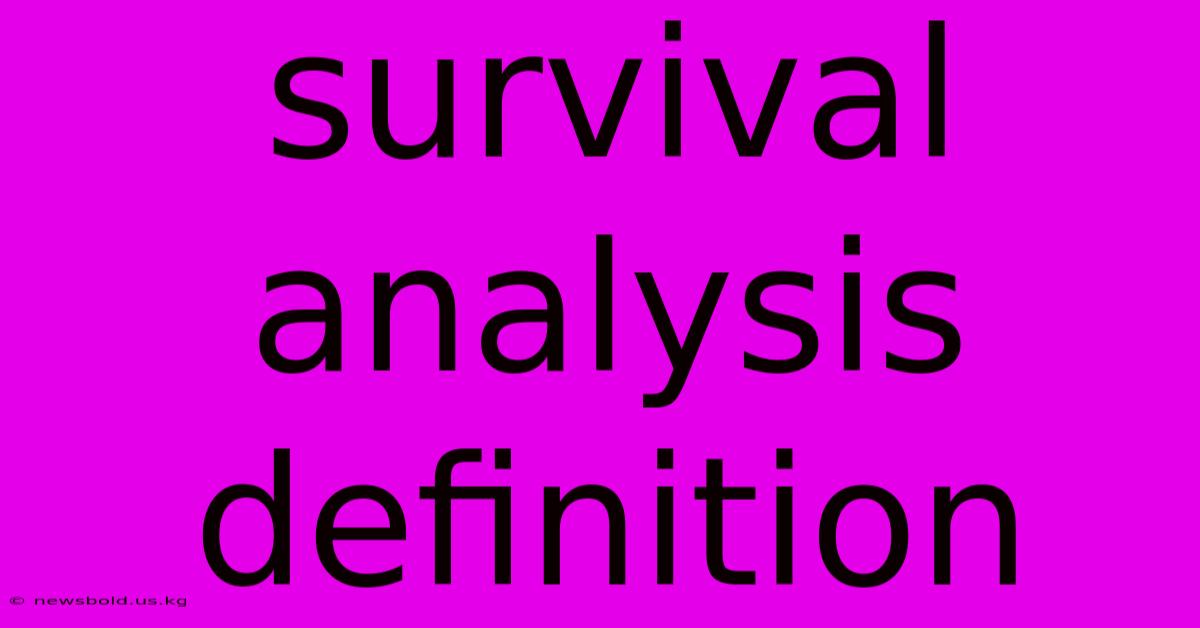
Thank you for taking the time to explore our website Survival Analysis Definition. We hope you find the information useful. Feel free to contact us for any questions, and don’t forget to bookmark us for future visits!
We truly appreciate your visit to explore more about Survival Analysis Definition. Let us know if you need further assistance. Be sure to bookmark this site and visit us again soon!
Featured Posts
-
Twenty Percent Rule Definition
Jan 08, 2025
-
Small Trader Definition
Jan 08, 2025
-
Trading Arcade Definition
Jan 08, 2025
-
Which Payday Loans Accept Chime
Jan 08, 2025
-
Separate Return Definition
Jan 08, 2025