Type 1 Error Definition False Positives And Examples
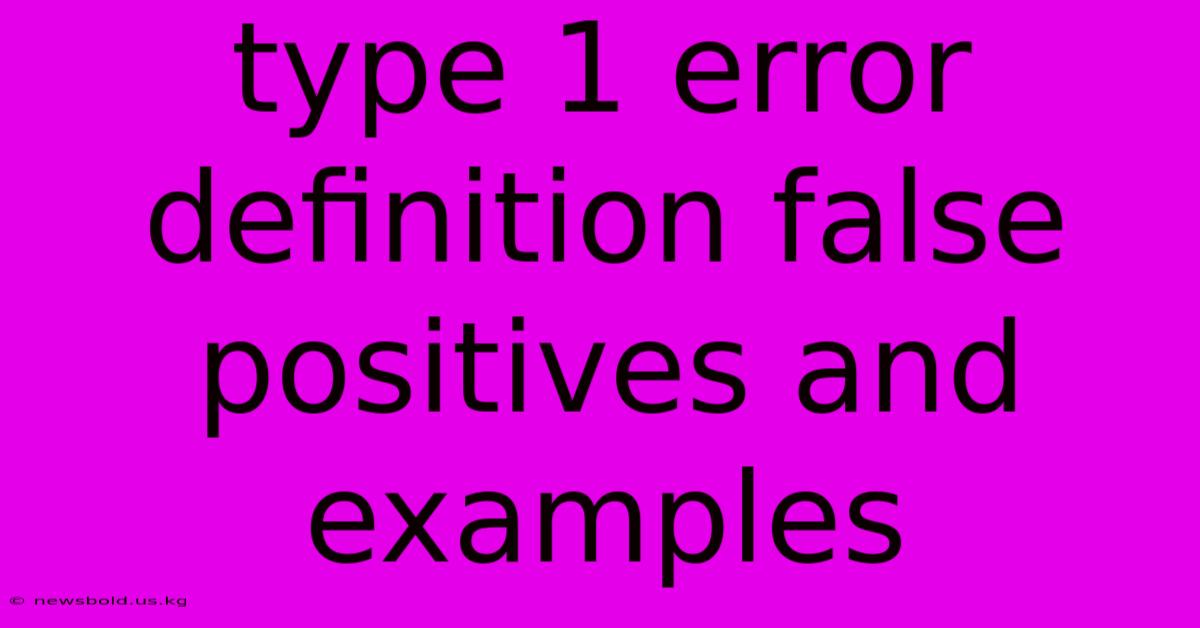
Discover more in-depth information on our site. Click the link below to dive deeper: Visit the Best Website meltwatermedia.ca. Make sure you don’t miss it!
Table of Contents
Unveiling Type I Errors: Understanding False Positives and Their Impact
What is the consequence of mistakenly rejecting a true null hypothesis? And how significant are the implications of such errors? This article delves into the critical concept of Type I error, commonly known as a false positive, exploring its definition, causes, consequences, and real-world examples across various fields.
Editor's Note: This comprehensive guide to Type I errors was published today to provide clarity and understanding of this crucial statistical concept.
Why It Matters & Summary: Understanding Type I errors is paramount for anyone interpreting data, conducting research, or making decisions based on statistical analysis. A false positive can lead to flawed conclusions, wasted resources, and potentially harmful actions. This article summarizes the definition of Type I error, its calculation (using significance levels), common causes (including issues with experimental design and data analysis), and illustrative examples across diverse fields like medicine, manufacturing, and finance. We'll also discuss strategies for mitigation. Relevant semantic keywords include: false positive, significance level, alpha level, p-value, null hypothesis, statistical significance, hypothesis testing, error rate, false alarm, Type I error rate, statistical error, data analysis, research methodology, experimental design, false discovery rate, Bayesian statistics.
Analysis: This guide synthesizes information from leading statistical textbooks, peer-reviewed research articles, and authoritative online resources to provide a comprehensive overview of Type I errors. The analysis focuses on providing practical examples and clear explanations to ensure accessibility for a wide audience, including researchers, students, and professionals in various fields needing to interpret statistical data.
Key Takeaways:
Point | Description |
---|---|
Type I Error Definition | Incorrectly rejecting a true null hypothesis (false positive). |
Significance Level (α) | Probability of committing a Type I error; typically set at 0.05 (5%). |
P-value | Probability of observing data as extreme as, or more extreme than, the obtained results if the null hypothesis is true. |
Consequences | Wasted resources, incorrect conclusions, potentially harmful actions. |
Mitigation Strategies | Careful experimental design, appropriate statistical tests, replication studies, adjusted p-values. |
Let's now transition to a deeper exploration of Type I errors.
Type I Error: A Deep Dive
Introduction
Type I error represents a fundamental concept in statistical hypothesis testing. It occurs when a researcher rejects the null hypothesis—the statement of no effect or no difference—when, in reality, the null hypothesis is true. The occurrence of a Type I error is directly influenced by the significance level (α), typically set at 0.05 or 5%. This means there's a 5% chance of making a Type I error if the null hypothesis is indeed true.
Key Aspects
The key aspects of Type I error involve understanding the null hypothesis, the alternative hypothesis, the p-value, and the significance level. A failure to correctly interpret or apply these concepts can significantly increase the likelihood of committing a Type I error.
Discussion
The connection between the significance level (α) and the probability of a Type I error is crucial. If α is set to 0.05, then 5% of the time, even if the null hypothesis is true, the researcher will incorrectly reject it. This doesn't mean the null hypothesis is false 5% of the time, but that the test has a 5% chance of leading to an incorrect rejection. This emphasizes the importance of carefully considering the significance level, balancing the risk of a Type I error with the risk of a Type II error (failing to reject a false null hypothesis). The choice of α depends on the context of the research; a lower α reduces the probability of a Type I error but increases the probability of a Type II error.
Significance Level (α) and the Probability of a Type I Error
Introduction
The significance level, often represented by α (alpha), is a critical parameter in hypothesis testing. It defines the threshold for rejecting the null hypothesis. This section explores the relationship between α and the probability of committing a Type I error.
Facets
- Role of α: α sets the acceptable probability of making a Type I error. A commonly used α level is 0.05 (5%).
- Examples: If α = 0.05, there's a 5% chance of rejecting a true null hypothesis. If α = 0.01, this chance is reduced to 1%.
- Risks and Mitigations: Setting α too low increases the risk of a Type II error (failing to reject a false null hypothesis). Setting α too high increases the risk of a Type I error. The optimal α depends on the context and the relative costs of each type of error.
- Impacts and Implications: The choice of α significantly impacts the interpretation of statistical results and influences the decision-making process.
Summary
The significance level (α) directly influences the likelihood of committing a Type I error. Careful consideration is essential to balance the risks associated with Type I and Type II errors, given the context of the research question and the potential implications of each type of error.
P-values and Their Interpretation
Introduction
The p-value is a pivotal component in hypothesis testing, providing information about the evidence against the null hypothesis. This section delves into the role of the p-value in relation to Type I errors.
Further Analysis
The p-value represents the probability of obtaining results as extreme as, or more extreme than, the observed data if the null hypothesis is true. If the p-value is less than or equal to the significance level (α), the null hypothesis is rejected. However, a low p-value doesn't automatically confirm the alternative hypothesis; it simply indicates that the observed data are unlikely if the null hypothesis were true. A common misconception is that the p-value represents the probability that the null hypothesis is true, which is incorrect.
Closing
Misinterpretations of p-values can directly lead to Type I errors. Understanding the p-value as a measure of evidence against the null hypothesis and not as the probability that the null hypothesis is true is vital for correct interpretation and to avoid committing a Type I error. A low p-value warrants further investigation and corroboration rather than immediate acceptance of the alternative hypothesis.
Examples of Type I Errors in Various Fields
- Medicine: A new drug is tested, and the results suggest it is effective in treating a disease (p < 0.05). However, further, larger-scale trials reveal the drug is ineffective; the initial positive result was a Type I error.
- Manufacturing: A quality control test indicates that a batch of products has a defect rate exceeding the acceptable limit. However, a more thorough examination reveals no defects; the initial test result was a false positive.
- Finance: A fraud detection system flags a transaction as potentially fraudulent. However, a subsequent investigation reveals the transaction was legitimate.
FAQ
Introduction
This section addresses frequently asked questions about Type I errors.
Questions
- Q: What is the difference between Type I and Type II errors? A: Type I error is incorrectly rejecting a true null hypothesis (false positive), while Type II error is failing to reject a false null hypothesis (false negative).
- Q: How can the probability of a Type I error be reduced? A: By lowering the significance level (α), increasing sample size, and using more powerful statistical tests.
- Q: Is a p-value of 0.049 statistically significant? A: Yes, if the significance level (α) is 0.05, a p-value of 0.049 is considered statistically significant, meaning the null hypothesis is rejected.
- Q: Can a Type I error lead to serious consequences? A: Yes, particularly in fields like medicine and safety engineering, where false positives can lead to incorrect treatments or unsafe products.
- Q: What is the relationship between power and Type I error? A: Increasing the power of a statistical test can reduce the probability of a Type II error without necessarily increasing the probability of a Type I error.
- Q: How does sample size impact Type I error? A: Larger sample sizes generally lead to more reliable results and reduce the likelihood of committing both Type I and Type II errors.
Summary
Understanding Type I errors requires understanding the underlying statistical concepts and their implications. This FAQ section highlights some critical points for better comprehension.
Tips for Avoiding Type I Errors
Introduction
This section provides practical tips for minimizing the risk of committing a Type I error.
Tips
- Choose an appropriate significance level (α): Carefully consider the relative costs of Type I and Type II errors when setting α.
- Employ rigorous experimental design: Ensure the study is well-designed to minimize confounding variables and biases that could lead to false positive results.
- Use appropriate statistical tests: Select statistical tests that are suitable for the type of data and research question.
- Perform multiple analyses cautiously: Avoid conducting numerous statistical tests without adjusting for multiple comparisons, which inflates the probability of a Type I error.
- Replicate findings: Conduct independent replication studies to confirm initial results and rule out the possibility of a Type I error.
- Consider effect sizes: Focus not only on statistical significance but also on the magnitude of the effect. A statistically significant result with a small effect size might be less meaningful.
- Utilize Bayesian methods: Bayesian approaches offer a framework for incorporating prior knowledge and updating beliefs based on new evidence, potentially reducing the reliance on p-values alone.
Summary
By following these tips, researchers can significantly reduce the probability of committing a Type I error and increase the reliability of their findings.
Summary
This article has provided a comprehensive overview of Type I errors, examining their definition, causes, and consequences across various disciplines. Understanding Type I errors is vital for accurate data interpretation and informed decision-making.
Closing Message
The risk of Type I errors is inherent in statistical inference. By comprehending the underlying principles and employing the strategies outlined here, researchers and professionals can minimize this risk and make more robust and reliable inferences from data. Continued vigilance and a thorough understanding of statistical methodology are crucial in mitigating the impact of Type I errors.
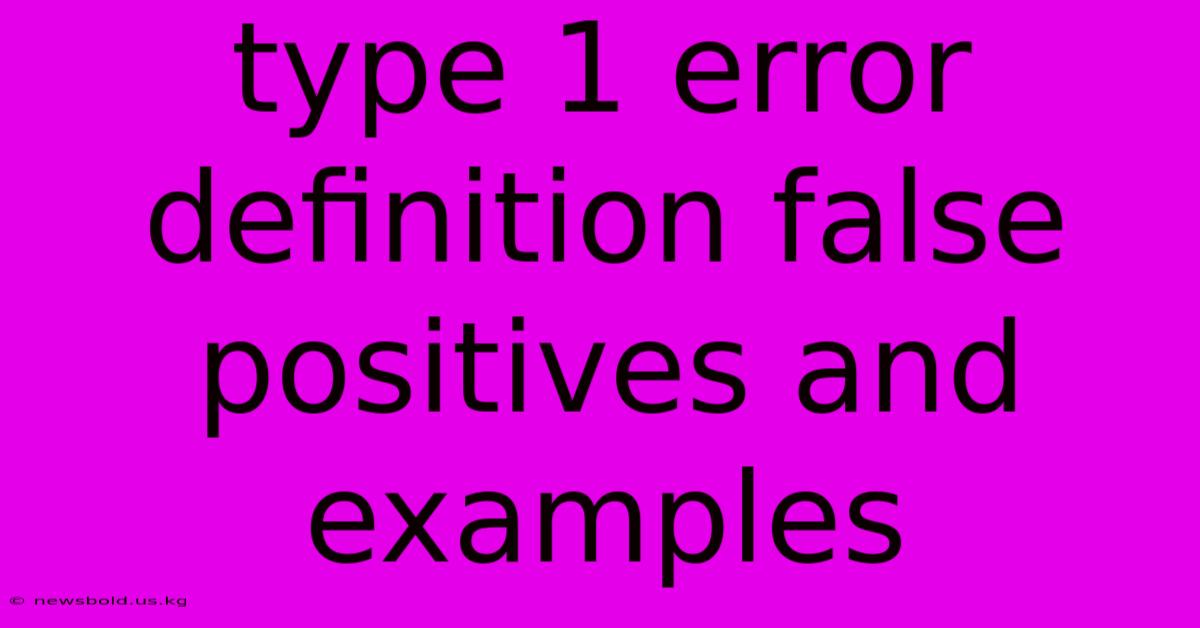
Thank you for taking the time to explore our website Type 1 Error Definition False Positives And Examples. We hope you find the information useful. Feel free to contact us for any questions, and don’t forget to bookmark us for future visits!
We truly appreciate your visit to explore more about Type 1 Error Definition False Positives And Examples. Let us know if you need further assistance. Be sure to bookmark this site and visit us again soon!
Featured Posts
-
Tax Rate Definition Effective Tax Rates And Tax Brackets
Jan 08, 2025
-
Availability Float Definition
Jan 08, 2025
-
Subordination Agreement Definition Purposes Examples
Jan 08, 2025
-
Software As A Service Saas Definition And Examples
Jan 08, 2025
-
What Is Conditional Reversal In Banking
Jan 08, 2025