What Is A Two Tailed Test Definition And Example
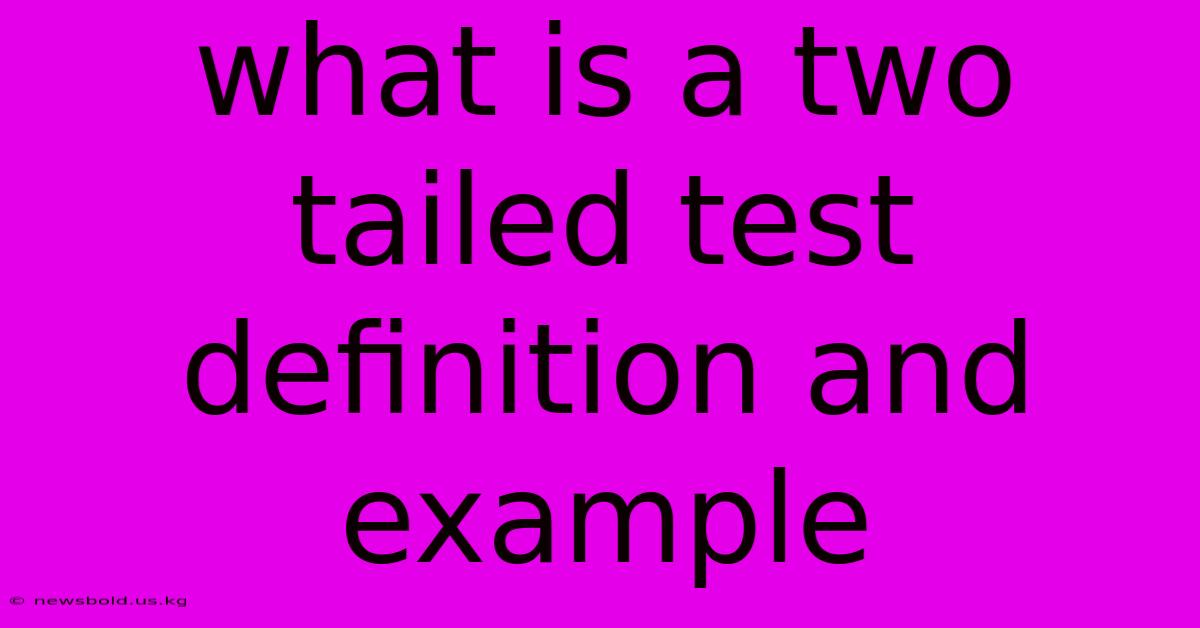
Discover more in-depth information on our site. Click the link below to dive deeper: Visit the Best Website meltwatermedia.ca. Make sure you don’t miss it!
Table of Contents
Unveiling the Two-Tailed Test: Definition, Examples & Applications
What distinguishes a situation where an outcome is simply different from expected versus demonstrably better or worse? This crucial distinction lies at the heart of statistical hypothesis testing, specifically within the framework of one-tailed versus two-tailed tests. This article delves into the definition and practical applications of two-tailed tests, offering clear explanations and illustrative examples.
Editor's Note: This comprehensive guide to two-tailed tests has been published today, providing readers with a clear understanding of this essential statistical concept.
Why It Matters & Summary
Understanding two-tailed tests is paramount for researchers and analysts across diverse fields. These tests assess whether a sample mean differs significantly from a hypothesized population mean in either direction—be it higher or lower. This is in contrast to a one-tailed test, which examines significance in only one direction. The summary below outlines key aspects:
- Hypothesis Testing: The foundation of inferential statistics, allowing generalizations from sample data to larger populations.
- Null Hypothesis (H₀): The statement being tested; often assumes no difference or effect.
- Alternative Hypothesis (H₁ or Hₐ): The statement accepted if the null hypothesis is rejected; for a two-tailed test, it suggests a difference in either direction.
- Significance Level (α): The probability of rejecting the null hypothesis when it's true (typically 0.05 or 5%).
- p-value: The probability of obtaining results as extreme as, or more extreme than, the observed results, assuming the null hypothesis is true.
Analysis
This analysis utilizes real-world examples to illustrate the application and interpretation of two-tailed tests. The examples are chosen to represent diverse scenarios where this statistical method proves invaluable. Data analysis involves comparing sample statistics (e.g., mean, proportion) to population parameters, utilizing appropriate statistical software (e.g., SPSS, R, Python) to compute p-values and draw inferences. The process includes clearly defining hypotheses, selecting an appropriate significance level, performing calculations, and carefully interpreting the results in context.
Key Takeaways
Feature | Description |
---|---|
Test Type | Two-Tailed Test |
Hypothesis | Tests for a difference in either direction (greater than or less than) |
Significance | Requires a p-value less than α/2 in each tail of the distribution to reject the null hypothesis |
Applications | Comparing means, proportions, or other parameters across groups, when the direction of the difference isn't specified beforehand |
Interpretation | A significant result indicates a statistically significant difference from the hypothesized value, without specifying the direction. |
Let's transition to a deeper exploration of the two-tailed test.
Two-Tailed Test: A Deep Dive
Introduction
The two-tailed test is a crucial tool in statistical inference. It allows researchers to determine if there is a statistically significant difference between a sample statistic and a hypothesized population parameter, without pre-assuming the direction of that difference. This is critical when prior knowledge doesn't suggest whether the effect should be positive or negative.
Key Aspects
- Symmetrical Distribution: The critical region (area where the null hypothesis is rejected) is split evenly between both tails of the probability distribution (e.g., normal, t-distribution).
- Non-directional Hypothesis: The alternative hypothesis does not specify the direction of the difference (e.g., H₁: μ ≠ μ₀, where μ is the population mean and μ₀ is the hypothesized mean).
- Increased stringency: Due to the split critical region, a larger absolute difference between sample and population means is needed to achieve statistical significance compared to a one-tailed test.
Discussion
The choice between a one-tailed and two-tailed test depends heavily on the research question. If there's prior evidence suggesting the direction of the effect, a one-tailed test might be appropriate. However, in many cases, particularly in exploratory research, the two-tailed test provides a more robust and unbiased approach, guarding against overlooking potential differences in the opposite direction of what was initially anticipated. The connection between the choice of test and the accuracy of conclusions is paramount for scientific rigor.
Example: Comparing Average Heights
Introduction
Let's consider a study comparing the average height of men and women. The null hypothesis (H₀) is that there is no difference in average height between men and women (μ_men = μ_women). The alternative hypothesis (H₁) is that there is a difference (μ_men ≠ μ_women). This is a two-tailed test because we are interested in detecting a difference in either direction – men being taller or women being taller.
Facets
- Roles: The sample means of men's and women's heights act as estimators for the population means. The t-test would be the appropriate statistical test.
- Examples: A sample of 100 men has a mean height of 175 cm and a sample of 100 women has a mean height of 163 cm.
- Risks & Mitigations: A Type I error (false positive) might occur if a small difference is incorrectly deemed statistically significant. Increasing the sample size minimizes this risk.
- Impacts & Implications: A significant result would imply a real difference in average height between men and women. This information could be valuable in fields such as clothing design, ergonomics, and healthcare.
Summary
In this example, a two-tailed test is essential as the direction of the height difference (if any) was not predetermined. The analysis focuses on whether any difference exists, irrespective of which group has the greater average height.
Example: Assessing Drug Effectiveness
Introduction
Suppose a pharmaceutical company is testing a new drug to lower blood pressure. The null hypothesis (H₀) states the drug has no effect on blood pressure (mean reduction = 0). The alternative hypothesis (H₁) states that the drug affects blood pressure (mean reduction ≠ 0). This is a two-tailed test because a significant increase in blood pressure would also be clinically important, even if unexpected.
Further Analysis
The study could involve measuring blood pressure before and after administering the drug to a sample of patients. A paired t-test could analyze the differences in blood pressure readings. If the p-value is below the significance level (e.g., 0.05), the null hypothesis is rejected, indicating a significant effect of the drug.
Closing
Two-tailed tests are particularly important in drug trials because an adverse reaction (increased blood pressure) is just as critical as a beneficial effect (decreased blood pressure). Understanding the implications of a two-tailed test is vital for responsible drug development and patient safety.
Information Table: Comparing One-Tailed and Two-Tailed Tests
Feature | One-Tailed Test | Two-Tailed Test |
---|---|---|
Hypothesis | Directional (greater than OR less than) | Non-directional (different from) |
Critical Region | One tail of the distribution | Both tails of the distribution |
Significance Level (α) | Entire α is in one tail | α/2 in each tail |
Power | Higher power if the effect is in the predicted direction | Lower power but more robust against unforeseen effects |
FAQ
Introduction
This section addresses common questions about two-tailed tests.
Questions
- Q1: When should I use a two-tailed test? A1: Use a two-tailed test when you're uncertain about the direction of the effect or want to detect a difference in either direction.
- Q2: What is the difference between a p-value of 0.03 and 0.07 in a two-tailed test with α=0.05? A2: 0.03 is statistically significant (reject H₀), while 0.07 is not (fail to reject H₀).
- Q3: Can I change from a two-tailed to a one-tailed test after seeing the results? A3: No, this is inappropriate and can bias the results. The directionality should be determined before the analysis.
- Q4: What if my p-value is exactly equal to my significance level? A4: In practice, it's best to consider the context and maybe collect more data to form a clearer conclusion.
- Q5: How do I calculate the p-value for a two-tailed test? A5: Statistical software packages automatically calculate this based on your data and chosen test statistic.
- Q6: Is a two-tailed test always better than a one-tailed test? A6: Not necessarily. A one-tailed test is more powerful if you are certain about the direction of the effect, but it risks missing an effect in the other direction.
Summary
Careful consideration of your research question and the nature of the expected effect dictates the most appropriate type of hypothesis test.
Tips for Conducting Two-Tailed Tests
Introduction
This section offers practical advice for implementing two-tailed tests effectively.
Tips
- Clearly Define Hypotheses: State your null and alternative hypotheses precisely before starting the analysis.
- Choose the Appropriate Test: Select the statistical test relevant to your data type (e.g., t-test, z-test, chi-squared test).
- Understand Your Data: Check for normality and outliers, which might influence the validity of your results.
- Interpret p-values Carefully: Don't solely rely on whether the p-value is above or below α; consider the effect size and the practical significance of your findings.
- Report Results Transparently: Clearly document your methodology, including the choice of a two-tailed test and the reasons for this choice.
Summary
Following these tips will enhance the rigor and interpretability of your analysis using two-tailed tests.
Summary
This article has provided a comprehensive guide to understanding and utilizing two-tailed hypothesis tests. From defining its core principles to illustrating its applications with real-world examples, it has emphasized its role in drawing valid and robust conclusions from statistical data. By carefully considering the choice between one-tailed and two-tailed tests, researchers can improve the reliability and integrity of their findings.
Closing Message
The mastery of two-tailed tests is a vital skill for anyone involved in data analysis and research. By understanding its implications and applying it correctly, one can navigate the complexities of statistical inference with greater confidence, ultimately leading to more informed decisions and a deeper understanding of the phenomena under investigation. Continue to explore advanced statistical techniques to further enhance your analytical capabilities.
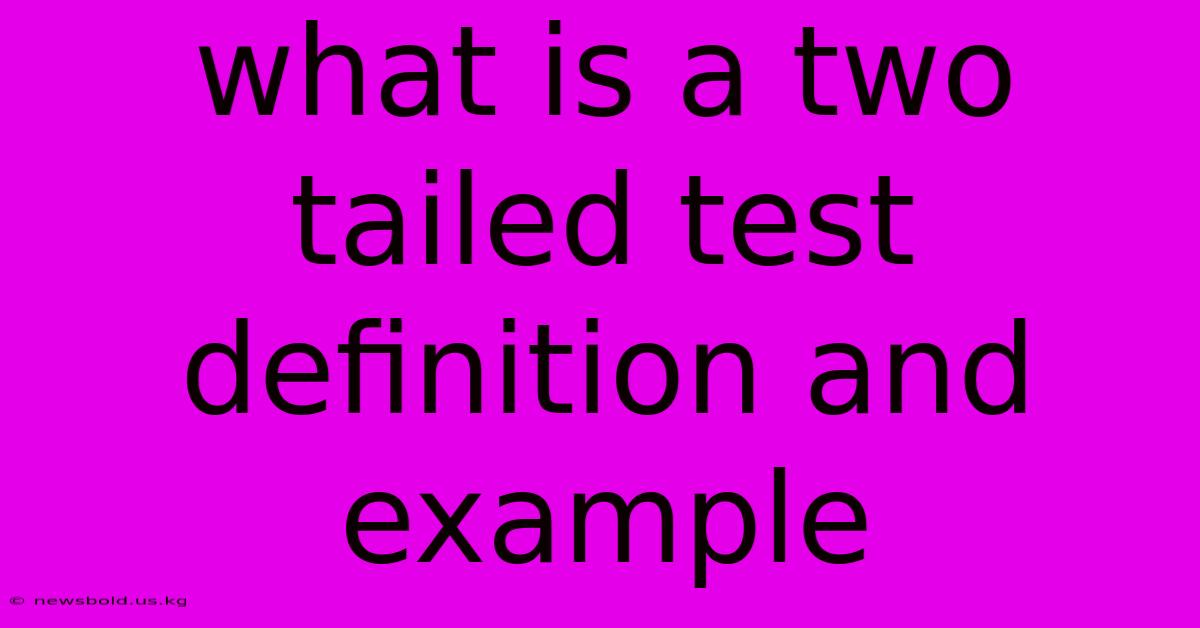
Thank you for taking the time to explore our website What Is A Two Tailed Test Definition And Example. We hope you find the information useful. Feel free to contact us for any questions, and don’t forget to bookmark us for future visits!
We truly appreciate your visit to explore more about What Is A Two Tailed Test Definition And Example. Let us know if you need further assistance. Be sure to bookmark this site and visit us again soon!
Featured Posts
-
What Is Asset Protection Trust Apt Definition And Purpose
Jan 08, 2025
-
What Is An Authorized Participant Definition Examples Benefits
Jan 08, 2025
-
What Is Basis In Accounting
Jan 08, 2025
-
Value Of Risk Vor Definition
Jan 08, 2025
-
Thomson First Call Definition
Jan 08, 2025