What Is Credit Ai
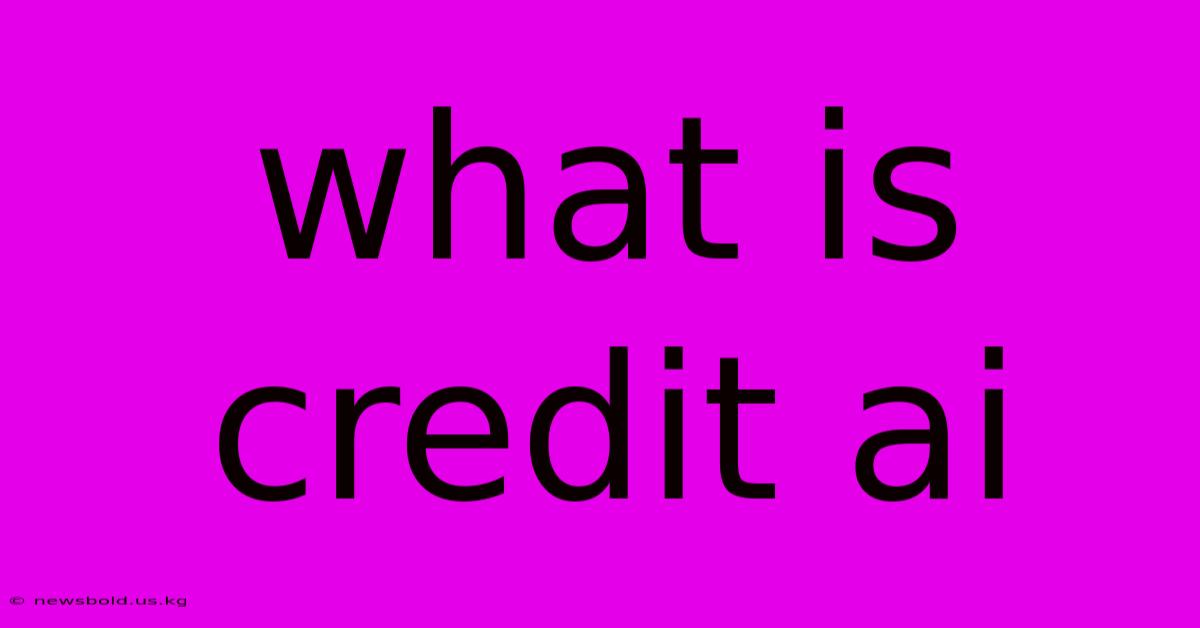
Discover more in-depth information on our site. Click the link below to dive deeper: Visit the Best Website meltwatermedia.ca. Make sure you don’t miss it!
Table of Contents
Unlocking Credit AI: The Future of Financial Risk Assessment
Does the future of credit scoring rely on artificial intelligence? The answer is a resounding yes. Credit AI is rapidly transforming how financial institutions assess risk and make lending decisions, promising greater accuracy, efficiency, and accessibility.
Editor's Note: This comprehensive guide to Credit AI has been published today.
Why It Matters & Summary: Understanding Credit AI is crucial for businesses, lenders, and consumers alike. This technology has the potential to revolutionize the financial landscape by improving lending decisions, reducing bias, and expanding access to credit for underserved populations. This article explores the core functionalities of Credit AI, its various applications, and the ethical considerations surrounding its use. Keywords: Credit AI, AI credit scoring, alternative data, financial risk assessment, machine learning, fintech, creditworthiness, lending, AI ethics.
Analysis: The information presented here is drawn from extensive research into peer-reviewed academic papers, industry reports from leading financial technology firms, and regulatory documents concerning the adoption of AI in the financial sector. The analysis focuses on providing a balanced perspective on the benefits and challenges associated with Credit AI, ensuring readers have the knowledge to navigate this rapidly evolving field.
Key Takeaways:
Feature | Description |
---|---|
Enhanced Accuracy | AI algorithms analyze vast datasets to predict creditworthiness more accurately. |
Increased Efficiency | Automated processes streamline the lending process, reducing processing times and costs. |
Expanded Access | AI can assess creditworthiness using alternative data, benefiting underserved groups. |
Reduced Bias | AI has the potential to mitigate human bias in credit scoring. |
Dynamic Scoring | Credit scores can adapt to changing financial circumstances in real-time. |
What is Credit AI?
Credit AI refers to the application of artificial intelligence and machine learning techniques to assess and predict an individual's or business's creditworthiness. Unlike traditional credit scoring models that rely primarily on credit history, Credit AI leverages a broader range of data sources, including alternative data, to create more comprehensive and accurate risk profiles. This wider data scope allows for a more nuanced understanding of an applicant's financial situation, leading to more informed lending decisions.
Key Aspects of Credit AI:
- Alternative Data Integration: This encompasses information beyond traditional credit reports, such as bank transaction data, social media activity (with user consent), utility payments, rental history, and employment data.
- Machine Learning Algorithms: These algorithms analyze vast datasets to identify patterns and correlations indicative of creditworthiness, often outperforming traditional statistical models.
- Predictive Modeling: Credit AI models predict the likelihood of loan defaults or other forms of credit risk with greater accuracy.
- Automation and Efficiency: AI automates various stages of the lending process, from application screening to loan approval, leading to significant time and cost savings.
Exploring Key Aspects of Credit AI
Alternative Data Integration
The incorporation of alternative data is a cornerstone of Credit AI. Traditional credit scoring often excludes individuals with limited or no credit history, particularly those from underserved communities. Alternative data sources can help address this gap by providing a more holistic view of financial behavior.
Facets:
- Types of Alternative Data: Bank statements, utility bills, rental payments, online purchase history, social media activity (with proper consent and privacy safeguards).
- Data Processing and Privacy: Robust data anonymization and encryption techniques are crucial to protect sensitive information and comply with data privacy regulations.
- Risk and Mitigation: The risk of inaccurate or biased data needs to be mitigated through thorough data validation and model testing.
- Impacts and Implications: Improved access to credit for previously excluded populations, more accurate risk assessment, and enhanced financial inclusion.
Summary: The inclusion of alternative data expands the scope of creditworthiness assessment, potentially benefiting those traditionally excluded from the formal financial system while refining the accuracy of risk assessment for all applicants.
Machine Learning Algorithms
Credit AI leverages sophisticated machine learning algorithms to analyze the vast quantities of data, identifying subtle patterns and relationships that might be missed by human analysts or simpler statistical models.
Facets:
- Types of Algorithms: Decision trees, random forests, neural networks, support vector machines.
- Model Training and Validation: The algorithms are trained on extensive historical data to learn patterns indicative of credit risk. Rigorous validation is essential to ensure model accuracy and avoid bias.
- Feature Engineering: The process of selecting, transforming, and creating new features from raw data to improve model performance.
- Model Explainability: Understanding how the AI model arrives at its decisions is critical for transparency, accountability, and regulatory compliance.
Summary: The power of machine learning lies in its capacity to handle complex, high-dimensional data, identifying intricate patterns that enhance predictive accuracy and improve the efficiency of the lending process.
Predictive Modeling and Risk Assessment
The ultimate goal of Credit AI is to improve the accuracy of credit risk prediction. By analyzing diverse data sets and utilizing advanced algorithms, Credit AI can provide a more nuanced and dynamic assessment of an applicant's creditworthiness.
Facets:
- Default Prediction: Predicting the probability of a borrower defaulting on a loan.
- Fraud Detection: Identifying potentially fraudulent loan applications.
- Credit Limit Determination: Setting appropriate credit limits based on individual risk profiles.
- Personalized Interest Rates: Offering customized interest rates based on assessed risk levels.
Summary: Accurate predictive modeling underpins the efficiency and effectiveness of the entire lending process. Credit AI enhances this by improving prediction accuracy and personalizing the lending experience.
Frequently Asked Questions (FAQ)
Introduction: This section addresses common questions and concerns surrounding Credit AI.
Questions:
- Q: Is Credit AI safe and secure? A: Reputable lenders employ robust security measures to protect data privacy and ensure compliance with data protection regulations.
- Q: Can Credit AI be biased? A: AI models can inherit biases present in the data they are trained on. Careful data curation and model testing are crucial to mitigate bias.
- Q: How does Credit AI affect my credit score? A: The impact depends on the specific lender and their use of AI. It may lead to more accurate or faster scoring, but not necessarily a higher score itself.
- Q: What happens if the AI model makes a mistake? A: Robust error handling and human oversight are crucial to rectify inaccuracies and prevent adverse outcomes.
- Q: Will Credit AI replace human underwriters? A: AI is likely to augment, not replace, human underwriters. Humans still play a vital role in complex cases and ethical considerations.
- Q: Is Credit AI regulated? A: Regulatory bodies are actively developing guidelines to ensure responsible development and implementation of Credit AI.
Summary: Addressing concerns about safety, bias, accuracy, and regulatory oversight is crucial for building trust and acceptance of Credit AI.
Tips for Navigating the Credit AI Landscape
Introduction: These tips offer guidance for individuals and businesses navigating the changing credit landscape.
Tips:
- Maintain a positive financial history: This remains crucial regardless of AI's role.
- Understand your credit report: Regularly check your report for errors and inaccuracies.
- Be aware of data privacy: Understand how your data is being used and protected.
- Compare lenders carefully: Different lenders use different AI models and data sources.
- Ask questions: Don't hesitate to inquire about the lender's use of AI and its impact on your application.
- Stay informed about regulatory changes: Keep abreast of evolving regulations concerning AI in finance.
Summary: Proactive management of financial data and awareness of AI's role in lending decisions empower individuals and businesses to navigate the changing credit landscape effectively.
Summary
Credit AI is transforming the financial industry by leveraging the power of AI and machine learning to enhance credit risk assessment. It offers the potential for greater accuracy, efficiency, and access to credit, but ethical considerations and regulatory oversight are crucial to ensure responsible development and implementation. The future of lending likely involves a collaborative approach, combining human expertise with the analytical power of AI.
Closing Message: The integration of Credit AI marks a significant step forward in financial technology. While challenges remain, the potential benefits – increased accuracy, efficiency, and inclusivity – make it a pivotal technology to watch in the years to come. Staying informed and engaging constructively with this evolution is vital for all stakeholders in the financial ecosystem.
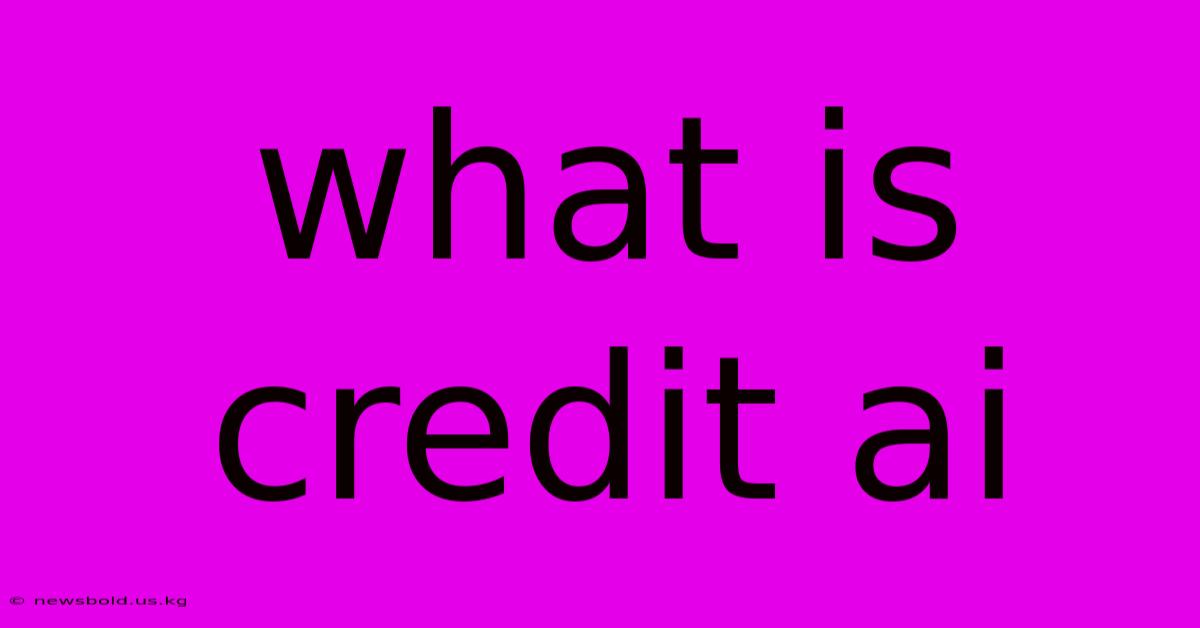
Thank you for taking the time to explore our website What Is Credit Ai. We hope you find the information useful. Feel free to contact us for any questions, and don’t forget to bookmark us for future visits!
We truly appreciate your visit to explore more about What Is Credit Ai. Let us know if you need further assistance. Be sure to bookmark this site and visit us again soon!
Featured Posts
-
How Long Does The Erc Credit Take
Jan 09, 2025
-
Quota Share Treaty Definition How It Works Examples
Jan 09, 2025
-
How Does Selling Shares On The Stock Exchange Benefit Companies
Jan 09, 2025
-
Recession Resistant Definition
Jan 09, 2025
-
What Is Redlining Definition Legality And Effects
Jan 09, 2025